Comprehensive Exploration of Databanks
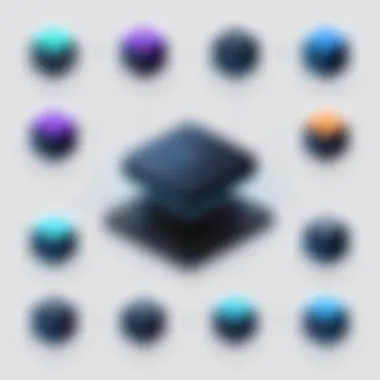
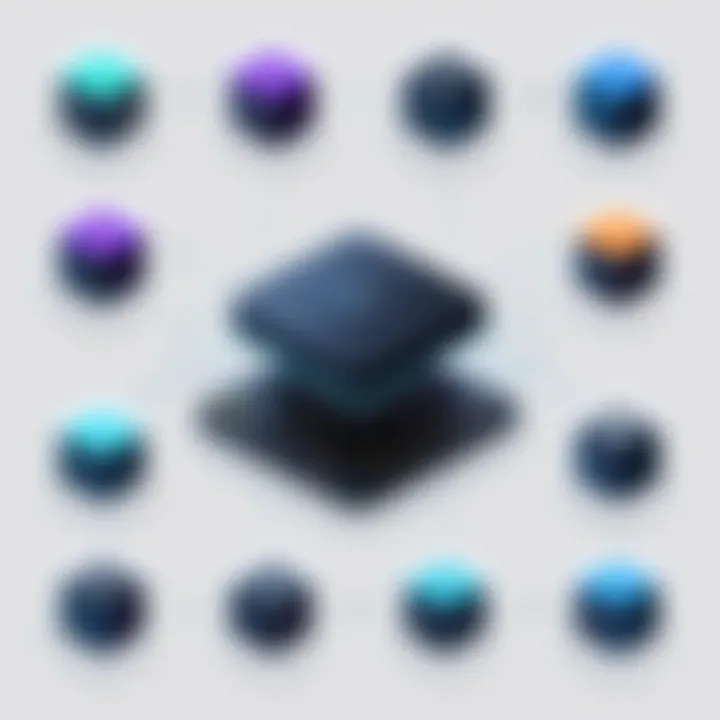
Intro
In today's fast-paced and data-driven environment, understanding databanks is crucial for businesses and organizations. The sheer volume of data generated daily necessitates effective storage and management solutions. Databanks serve as repositories for these large datasets, enabling organizations to derive insights, enhance productivity, and streamline operations.
The significance of databanks extends beyond mere storage. They play a pivotal role in decision-making processes across various sectors, from finance to healthcare and beyond. This article will provide a detailed examination of databanks, highlighting their features, types, pricing models, and applications. Additionally, we will delve into the ethical and security considerations surrounding databank implementation. By the end, readers will have a clearer understanding of how to leverage databanks to their advantage.
Key Features
Overview of Features
Databanks have several key features that make them indispensable in modern data management. These include:
- Scalability: Databanks are designed to handle growing amounts of data without compromising performance.
- Accessibility: Users can easily access, query, and retrieve information stored within databanks, facilitating quicker decision-making.
- Integration: They seamlessly integrate with various data processing tools, enabling organizations to utilize their existing infrastructure efficiently.
- Data Security: Implementing strong security measures ensures that sensitive data remains protected from unauthorized access and breaches.
Unique Selling Propositions
When considering databanks, certain unique selling propositions should be evaluated:
- Cost Efficiency: By automating data storage and retrieval processes, organizations can reduce operational costs significantly.
- Enhanced Data Analysis: Databanks allow for advanced analytics capabilities, helping organizations discover patterns and gain valuable insights from data.
- User-Centric Design: Many databanks focus on user experience, ensuring that their interfaces are intuitive and easy to navigate.
In summary, the key features of databanks contribute to their growing importance in today's data landscape. Understanding these features can aid organizations in selecting the best solutions tailored to their specific needs.
Pricing Models
Different Pricing Tiers
Various pricing models exist for databanks, depending on their scale and functionality. Common pricing tiers include:
- Subscription-Based: Monthly or yearly fees for access to databank services. This model suits organizations that prefer predictable costs.
- Pay-As-You-Go: This model charges based on usage, allowing businesses to only pay for the resources they actually use.
- Flat Fee: A one-time payment for accessing the databank, suitable for smaller projects or one-time analyses.
ROI and Cost-Benefit Analysis
When investing in databanks, organizations must consider the return on investment and perform a cost-benefit analysis. The potential benefits include:
- Increased efficiency in data handling.
- Reduced time spent on data retrieval tasks.
- Improved decision-making supported by accurate and timely data.
Ultimately, choosing the right databank model can lead to substantial long-term advantages for any organization.
Intro to Databanks
Databanks serve as crucial instruments in todayβs data-centric world. Their relevance spans multiple sectors, impacting business operations and decision-making. Understanding their definition, historical significance, and the value they offer is essential for stakeholders aiming to optimize data utilization.
Databanks not only store data but also provide frameworks for managing, retrieving, and analyzing information. They streamline processes and enable organizations to make informed decisions based on accurate data analysis. As businesses continue to navigate complex data landscapes, databanks act as the backbone for efficient data management.
Defining Databanks
A databank is a structured repository that holds data organized for easy access and analysis. It encompasses a variety of systems designed to store, retrieve, and manage data effectively. Databanks can range from simple databases to extensive systems incorporating various technologies such as cloud storage and artificial intelligence.
The classification of databanks can follow different criteria. For instance, some databanks are public, offering access to a broad audience, while others are private, serving specific organizational needs. Organizational databanks, project-specific databanks, and commercial databanks all fall within this category, showcasing their diverse functionality in different contexts.
In todayβs digital landscape, databanks are integral for companies seeking to leverage data for competitive advantages. They enable organizations to draw insights from vast amounts of information, enhancing decision-making and operational efficiency.
Historical Context
The concept of databanks has evolved significantly over the decades. Initially, data storage was cumbersome, often relying on physical mediums such as paper or magnetic tapes. The advent of computers brought about significant changes, leading to the creation of databases.
In the 1970s, the first relational database management systems (RDBMS) emerged, revolutionizing how data was stored and accessed. This advancement cast a spotlight on databanks as essential tools for businesses seeking to organize their data.
The rise of the internet and cloud computing further transformed databanks. Now, organizations can access, share, and analyze data in real-time, breaking geographical barriers and fostering global collaboration.
"Data is a precious thing and will last longer than the systems themselves."
- Tim Berners-Lee
This trajectory of development has established databanks not merely as storage solutions but as pivotal components driving innovation and strategic initiatives across industries.
Categories of Databanks
Understanding the categories of databanks is essential for grasping their diverse functionalities and applications. Each category serves specific purposes and addresses unique needs within various sectors. By recognizing these distinctions, decision-makers and IT professionals can choose the most suitable databank types that align with their organizational objectives.
Public Databanks
Public databanks are repositories of data that are freely accessible to the public. They play a vital role in promoting transparency and facilitating research, education, and innovation. Users can find various types of data, including demographic statistics, environmental data, and scientific research findings. Public databanks often originate from government agencies, academic institutions, and non-profit organizations.
Benefits of public databanks include:
- Accessibility: Information is available to anyone who seeks it, fostering equitable access to valuable data.
- Collaboration: Researchers and organizations can build upon existing data, leading to advancements in various fields.
- Transparency: As public entities handle these databanks, they serve to hold organizations and governments accountable.
However, users must examine the quality and reliability of the data provided. Not all public databanks are consistently updated or verified, which can impact the integrity of the findings derived from them. A conscious selection of sources is necessary for effective use.
Private Databanks
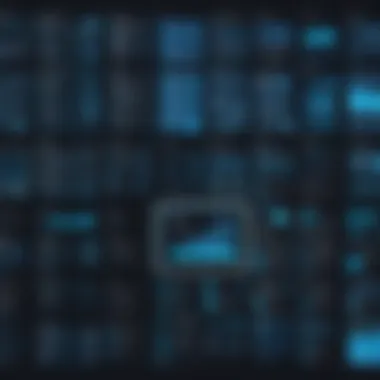
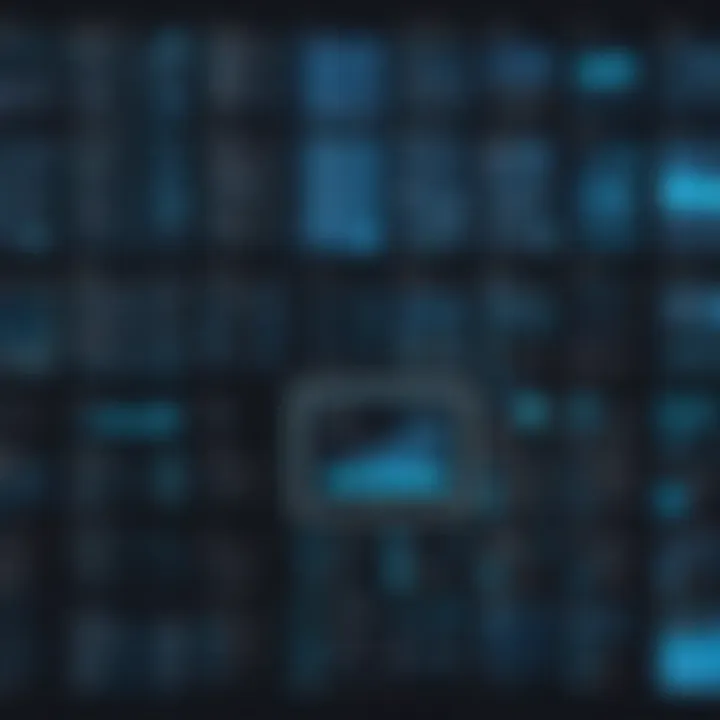
Private databanks are maintained by organizations for internal use. These databanks often house sensitive or proprietary information not intended for public access. Companies leverage private databanks to enhance data security, inform strategic decision-making, and streamline operations.
The advantages of private databanks include:
- Data Control: Organizations retain ownership and control of their data, mitigating risks related to unauthorized access.
- Tailored Solutions: Companies can customize their databanks to serve their specific needs and workflows.
- Enhanced Security: Unlike public databanks, private databanks employ strict security protocols to protect sensitive information.
Despite these benefits, private databanks also face challenges, such as significant costs for setup and maintenance. Organizations need skilled personnel to manage and analyze the data effectively, which can strain smaller entities.
Project-Specific Databanks
Project-specific databanks are created for particular projects or initiatives. They are designed to collect, store, and manage data pertinent to a particular objective, often involving collaboration between multiple stakeholders. Such databanks are prevalent in industries like healthcare, environmental studies, and research.
Key features of project-specific databanks include:
- Focused Data Collection: Data is collected with a targeted approach, ensuring relevancy to the project.
- Collaborative Efforts: These databanks facilitate partnerships among organizations, allowing for the pooling of resources and data sharing.
- Flexibility: They can be adjusted as projects evolve, accommodating changing requirements and goals.
On the downside, project-specific databanks may require substantial time and effort to create and maintain. Additionally, the data collected may not be as comprehensive or robust as that found in larger databanks, which could limit its applicability in broader contexts.
In summary, each category of databanks serves a distinct purpose, with unique advantages and considerations. Understanding these categories equips organizations with the knowledge to navigate the complex landscape of data management effectively. An informed choice regarding databank usage can significantly enhance data-driven decision-making and operational efficiency.
Core Functions of Databanks
Databanks serve as a crucial backbone for managing and utilizing data effectively. Their core functions are essential for businesses, organizations, and individuals who rely on data-driven decision-making. Understanding these functions can help stakeholders optimize their operations, improve performance, and enhance productivity. This section elaborates on the primary roles of databanks, focusing on data storage and management, retrieval and analysis, and reporting.
Data Storage and Management
Data storage and management refer to how information is organized, maintained, and secured within a databank. This function is fundamental for any data-driven entity. A well-structured databank allows for easy access to information while ensuring its integrity and reliability.
Key elements of data storage include:
- Centralized Storage: All data is stored in one location, simplifying management and access.
- Data Organization: Proper classification of data types enables efficient retrieval and analysis.
- Backup and Recovery: Regular updates and backups protect against data loss, ensuring business continuity.
Effective data management practices enhance operational efficiency. Businesses can avoid data silos, reduce redundancy, and improve data quality. Furthermore, with the rise of regulations such as GDPR, maintaining proper data management is vital to ensure compliance and protect user privacy.
Data Retrieval and Analysis
The retrieval of data is directly linked to the efficiency of operations. Data retrieval systems must allow users to access relevant information quickly. A robust databank will support various methods of data access, including querying, filtering, and sorting.
In-depth analysis of this data forms the basis of informed decision-making. Successful data retrieval and analysis contribute to:
- Enhanced Decision-Making: Real-time access to data enables quicker and more accurate decisions.
- Identifying Trends: Businesses can analyze historical data to identify patterns, informing strategy.
- Performance Tracking: Analysis tools help track key performance metrics to assess operational success.
Employing analytical tools like SQL or business intelligence platforms can escalate data retrieval capabilities. These technologies empower users with actionable insights, further integrating data into strategic planning and execution.
Data Reporting
Data reporting is the final pivotal function of databanks. It involves summarizing information and presenting it in an easily digestible format, facilitating transparency and strategic insights within an organization.
Critical aspects of data reporting include:
- Customizable Reports: Users should have the ability to tailor reports to fit specific informational needs.
- Visual Aids: Including charts and graphs can make data more comprehensible; visuals highlight trends instantly.
- Scheduled Reporting: Automating report generation saves time and ensures stakeholders receive timely updates.
Regular reporting aids in monitoring progress against objectives, making it easier to pivot strategies as necessary. For organizations, this contributes to fostering a culture of accountability and continuous improvement.
"Effective databank functions create a seamless flow of data, allowing organizations to adapt strategies with agility."
Technological Framework of Databanks
In the context of databanks, the technological framework is a crucial element influencing how data is stored, processed, and retrieved. An effective technological framework enables organizations to manage vast amounts of information efficiently. It combines hardware, software, and networking components to create a robust environment for data management. The right technology not only enhances performance but also ensures security and compliance with regulations.
Database Management Systems
Database Management Systems (DBMS) play a vital role in the operational structure of databanks. A DBMS allows users to create, retrieve, update, and manage data systematically. These systems provide an interface for users to interact with the data without needing to understand the complexities of the underlying architecture. Databanks utilize different types of DBMS, such as relational, NoSQL, and object-oriented systems, each with unique benefits.
- Relational DBMS (e.g., MySQL, PostgreSQL) organizes data into tables, making it easier to perform complex queries.
- NoSQL DBMS (e.g., MongoDB, Cassandra) offers flexibility in handling unstructured data, ideal for large-scale applications.
- Object-oriented DBMS allows data to be represented in the form of objects, enhancing the integration of data and operations.
Overall, the choice of a DBMS is essential as it directly affects data access speed, reliability, and scalability. When selecting a DBMS, organizations need to consider factors such as data types, user interactions, and expected growth. Ideally, the DBMS should be aligned with organizational goals to support data-driven decision-making processes.
Cloud-based Databanks
Cloud-based databanks represent a shift in how data is stored and managed. They rely on cloud computing technologies that enable data access over the internet rather than local servers. This framework offers compelling advantages for businesses.
- Scalability: Organizations can easily scale their storage options up or down based on demand without heavy investment in physical infrastructure.
- Cost Efficiency: Cloud services generally operate on a pay-as-you-go model, reducing upfront costs associated with physical databanks.
- Accessibility: Data can be accessed from virtually anywhere, facilitating remote work and collaboration.
However, while cloud-based databanks provide numerous benefits, they also come with challenges. Data security is paramount, as sensitive information could be exposed to potential threats. Additionally, organizations must ensure they comply with regulations when using cloud services to manage data. Selecting a reputable cloud service provider with strong security practices is crucial in mitigating these risks.
"The integration of cloud-based technology brings not just efficiency, but also a new dynamic in how organizations view and manage their databanks."
As such, a solid understanding of both DBMS and cloud-based solutions is integral for the effective management of databanks. This ensures that organizations can leverage data optimally to support business objectives.
The Role of Databanks in Business Intelligence
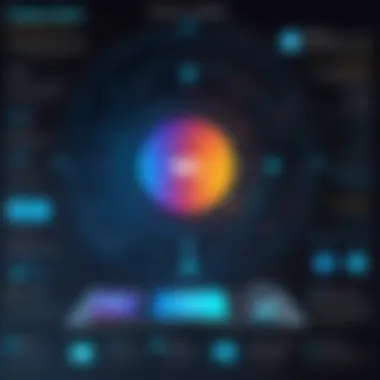
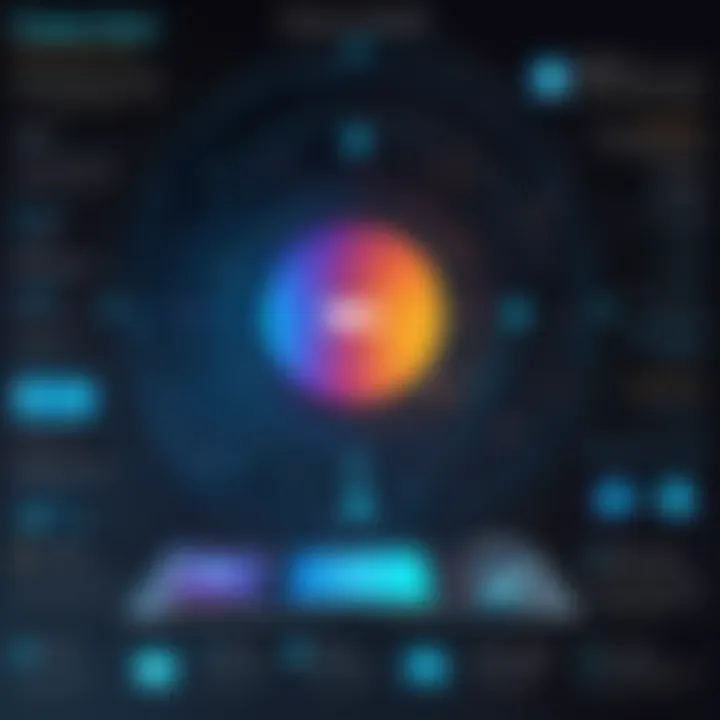
Databanks serve as the backbone for Business Intelligence (BI), facilitating informed decision-making. They gather, store, and manage vast amounts of data. This data is crucial for organizations aiming to gain a competitive edge. Effective use of databanks enables businesses to turn data into actionable insights. Without a robust databank, achieving thorough analysis of performance, customer behavior, and market trends becomes nearly impossible.
Data-Driven Decision Making
Data-driven decision making is a strategic approach where decisions are based on data analysis and interpretation. In this context, databanks play a pivotal role. Organizations that utilize databanks can make informed choices founded on real evidence rather than intuition or guesswork.
- Enhanced Accuracy: By relying on data stored within databanks, companies reduce the likelihood of errors in their decisions. Accurate data allows for realistic forecasting and planning.
- Informed Strategies: With proper data retrieval, businesses can develop strategies tailored to meet precise market demands. They can analyze customer preferences and adjust accordingly.
- Resource Optimization: Companies can allocate resources more efficiently. A databank can highlight which areas require investment and which can be minimized.
In the age of information, data-driven decision-making is not just advantageous; it is essential. Companies that leverage their databanks effectively often gain a distinct advantage over competitors who do not.
Performance Metrics
Measuring performance is critical for any organization. Databanks enable this by providing tools for performance metrics analysis.
- Key Performance Indicators (KPIs): Databanks can track various KPIs, which are essential for evaluating the success of business operations. For example, sales data over different periods can inform future sales strategies.
- Real-Time Analysis: The capability to analyze data in real time enhances an organization's responsiveness. For instance, if consumer interest shifts, businesses can adapt their strategies faster than those reliant on outdated data.
- Benchmarking: Organizations can use databanks to compare their performance against industry standards. This gives them insights into their relative position within the market.
Overall, databanks directly impact Business Intelligence by translating data into meaningful information. This transition facilitates smarter decision-making and better performance evaluation.
Applications of Databanks Across Industries
In a data-driven environment, databanks have emerged as pivotal assets across various sectors. Their ability to store, manage, and curate vast amounts of information allows organizations to enhance their operational efficiency and decision-making capabilities. As industries evolve, the application of databanks becomes increasingly specialized and integral, serving unique purposes that cater to the particular needs of each field. This section examines how databanks are employed in three prominent industries: healthcare, finance, and marketing and sales.
Healthcare
The healthcare sector relies heavily on databanks to streamline patient care, manage research data, and facilitate regulatory compliance. Databanks store electronic health records (EHRs), which contain comprehensive patient histories, treatment plans, and outcomes. By analyzing this data, healthcare providers can uncover patterns that lead to improved patient treatment protocols and faster diagnoses.
Moreover, research institutions use databanks to manage clinical trial data. This allows researchers to track participant responses, assess drug efficacy, and ensure adherence to ethical standards. The integration of databas into healthcare fosters collaboration among different professionals and improves patient outcomes through data sharing.
Additionally, databanks support public health initiatives. They can monitor outbreaks of diseases and track vaccination rates, helping officials respond quickly to potential health crises. This capability is crucial in todayβs world where health challenges can spread rapidly.
"Data is the new oil" - This statement highlights the significance of data in informing healthcare decisions and guiding policy-making in public health.
Finance
In finance, databanks play a critical role in risk management and investment analysis. Financial institutions utilize databanks to aggregate market data, customer transactions, and economic indicators. This data is crucial for assessing investment risks, forecasting trends, and ensuring compliance with regulations.
Databanks facilitate faster decision-making. For example, bankers can quickly retrieve client credit histories and transaction patterns, enabling them to approve loans and manage accounts effectively. Trading firms also leverage real-time data from databanks to execute trades efficiently, capitalizing on market fluctuations.
Furthermore, financial analysis depends heavily on historical data stored in databanks. Analysts often compare past performance with current market conditions to inform investment strategies and improve portfolio management.
Marketing and Sales
In the realms of marketing and sales, databanks offer invaluable insights into consumer behavior, preferences, and trends. Companies utilize databanks to collect and analyze comprehensive datasets, which enable them to segment customers effectively and target their marketing efforts.
With databanks, businesses can track campaign effectiveness and customer engagement in real-time. This immediate feedback loop allows for agile marketing strategies that can adapt to changing market conditions or consumer preferences.
Additionally, databanks empower sales teams with crucial information regarding potential leads. By analyzing past purchasing behavior and customer demographics, sales professionals can focus their efforts on high-value prospects. This streamlines the sales process, optimizes resource use, and enhances overall performance.
In sum, databanks are central to various industries. They drive efficiency, support strategic planning, and improve outcomes across healthcare, finance, and marketing and sales. As organizations continue to harness the power of data, the role of databanks will only expand.
Ethics and Data Governance in Databanks
Ethics and data governance are crucial elements in the management of databanks. As databanks grow in size and scope, the need for ethical considerations becomes increasingly significant. Ethical practices ensure that data is used responsibly, with respect for privacy and individual rights. In addition, effective governance frameworks help organizations comply with legal requirements and maintain trust among their stakeholders.
Data Privacy Considerations
Data privacy is paramount in the context of databanks. Organizations collect, store, and process vast amounts of personal data. Such data can include sensitive information about individuals. Therefore, safeguarding this data from unauthorized access and misuse is essential.
Key aspects of data privacy considerations include:
- Informed Consent: Individuals must be aware of how their data will be used. This is not just an ethical obligation but also a legal requirement in many jurisdictions.
- Data Minimization: Organizations should only collect data that is necessary for their operations. Excessive data collection raises risks and complicates governance.
- Transparency: Communication with stakeholders about data practices builds trust. It is vital for organizations to clearly articulate data handling practices.
Implementing strong data privacy measures can enhance the reputation of organizations and foster customer loyalty. As a result, the integration of privacy protections in databanks is imperative.
Compliance Frameworks
Compliance frameworks are essential for managing data within databanks. They help ensure that organizations adhere to relevant regulations and best practices. Failing to comply with these frameworks can lead to serious legal repercussions and reputational harm.
Some critical compliance frameworks include:
- GDPR (General Data Protection Regulation): This regulation governs data protection and privacy in the European Union. It lays out strict guidelines on data collection, processing, and sharing.
- HIPAA (Health Insurance Portability and Accountability Act): This U.S. regulation ensures the protection of patient data in the healthcare sector. It mandates strict data handling practices for personal health information.
- CCPA (California Consumer Privacy Act): This act provides California residents with rights regarding their personal data and compels businesses to comply with data privacy rights.
Organizations must regularly review their compliance status. This helps in adjusting to changes in laws and technology. Effective compliance not only protects organizations from legal issues but also contributes to a data governance culture that values ethical practices.
Therefore, ethics and data governance should not be afterthoughts. They must be integral to the strategy and operations surrounding databanks.
Challenges in Databank Management
Managing databanks comes with various challenges that can significantly impact their effectiveness and usability. These challenges need careful attention from IT professionals, business owners, and decision-makers to ensure proper functionality and secure operations. Understanding these difficulties allows organizations to anticipate potential pitfalls and implement appropriate measures. The two major challenges in databank management are data quality issues and security vulnerabilities.
Data Quality Issues
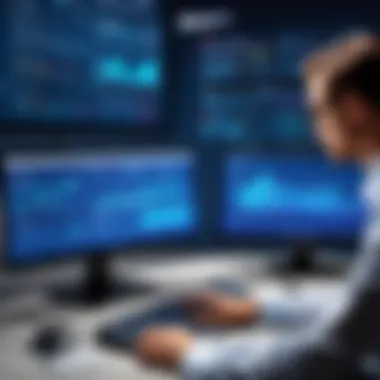
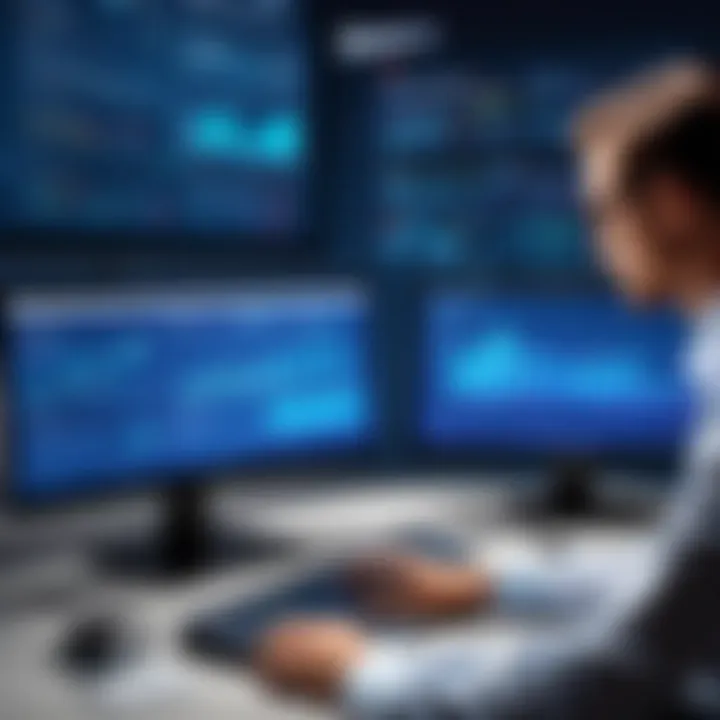
Data quality is crucial for the overall success of a databank. Poor data quality can lead to incorrect conclusions and misguided decisions. When the data stored in a databank is inaccurate or incomplete, it can directly impact analyses, reporting, and decision-making processes.
Organizations often struggle with the following aspects:
- Data entry errors: Manual data entry often leads to mistakes. Typos, misclassifications, and incomplete forms can introduce significant inaccuracies.
- Inconsistent data sources: If data is collected from multiple sources without standardization, it may result in discrepancies. Different formats, terminologies, or measurement units can distort analyses.
- Outdated information: Regularly updated data is essential for relevancy. Stale data can skew reports, making them less effective in reflecting current conditions.
To combat these issues, organizations should prioritize data cleansing processes and establish stringent data governance policies. Institutions must also implement regular audits to ensure data integrity and formulate comprehensive training programs for employees involved in data handling.
Security Vulnerabilities
Security vulnerabilities pose a serious threat to databanks, as unauthorized access or data breaches can have severe consequences. Organizations operate in a landscape where data is a valuable asset, necessitating robust security measures.
Common security challenges include:
- Unauthorized Access: Without strong access controls and authentication mechanisms, sensitive data can be accessed by unauthorized individuals, leading to potential data leaks and breaches.
- Data Encryption: Inadequate encryption practices expose databanks to risks, especially when data is transmitted over networks. Encryption is essential to protect data from eavesdropping and interception.
- Insider Threats: Employees with legitimate access can also pose risks. Malicious actions by insiders or careless handling of data can result in security breaches.
To ensure the safety of databanks, companies must adopt comprehensive security strategies. This entails investing in advanced encryption techniques, conducting regular security audits, and implementing training programs to educate employees about data security best practices.
"Effective databank management requires a proactive approach to both data quality and security threats. Addressing these issues fortifies organizational operations and bolsters trust in data-driven decisions."
By recognizing these challenges, organizations can devise practical strategies that enhance databank performance and security, ultimately leading to improved decision-making and operational success.
Future Trends in Databank Technology
The landscape of databanks is changing rapidly, driven by advancements in technology and the increasing need for efficient data management. Understanding the future trends in databank technology is important. These trends not only influence how data is stored, shared, and maintained, but also dictate the competitive advantage businesses can gain. The integration of technologies like artificial intelligence and blockchain is at the forefront of these trends. Companies need to adapt to these changes to stay relevant.
Artificial Intelligence Integration
Artificial Intelligence (AI) is becoming a pivotal element in the realm of databanks. The integration of AI enables better data processing, analytics, and management. With AI algorithms, databanks can handle vast amounts of data more efficiently, providing insights that are not easily attainable through manual processes.
Here are some benefits of AI in databanks:
- Enhanced Data Analysis: AI can identify patterns and trends in complex datasets that would be challenging for human analysts to detect.
- Predictive Capabilities: Businesses can leverage AI tools for predicting future behaviors or trends based on historical data.
- Automation: Routine tasks such as data cleaning and organization can be automated, allowing professionals to focus on strategic decision-making rather than data handling.
This integration raises several considerations. Companies must invest in training staff to work effectively with AI technologies. Additionally, ethical concerns around data handling and privacy must be addressed as AI becomes more integrated into databank operations.
Blockchain Applications
Blockchain technology is another key trend influencing databanks. Its distributed ledger structure brings a new level of security and transparency to data storage. This technology allows for secure and verified data transactions, which is crucial in sectors like finance and healthcare.
The benefits of applying blockchain in databanks include:
- Increased Security: The decentralized nature of blockchain minimizes the risk of unauthorized data access.
- Data Integrity: Every transaction is recorded and cannot be altered, ensuring that the data remains trustworthy.
- Smart Contracts: Automated contracts can execute transactions or data access based on predefined conditions, enhancing efficiency.
Companies considering blockchain must contemplate the complexity and costs associated with implementing this technology. Understanding both the advantages and barriers is necessary for effective utilization in databanks.
"The future of databank technology hinges on the ability to innovate while addressing ethical and practical concerns."
In summary, the future of databank technology is being shaped by advancements in AI and blockchain. These trends enhance data management capabilities, strengthen security, and offer strategic insights for businesses. Failing to adapt to these developments can hinder competitive positioning in an increasingly data-driven marketplace.
Best Practices for Effective Databank Usage
When discussing databanks, it is crucial to understand that their effective usage is not merely about having the right technology. Best practices in databank usage ensure that organizations can fully leverage their data assets to make informed decisions and improve operational efficiency. Following effective strategies will maximize the benefits associated with databanks.
Data Management Strategies
Creating a robust data management strategy is essential. This encompasses how data is collected, processed, stored, and secured. Key strategy elements include:
- Data Quality Management: Ensure data accuracy and consistency by routinely cleansing the databank. Eliminating duplicates and correcting errors can significantly enhance the usability of data.
- Access Control: Implementing strict access mechanisms is fundamental. Data should be accessible only to authorized personnel, which helps maintain confidentiality and integrity.
- Data Classification: Classifying data based on its sensitivity and importance allows for tailored security measures and efficient retrieval processes.
Additionally, organizations should establish clear protocols for data backup and recovery. Regular backups protect against data loss, while a recovery plan is essential for handling potential data breaches.
Evaluating Databank Solutions
Evaluating databank solutions involves a careful consideration of different factors that align with the business requirements. Here are several criteria to consider:
- Scalability: As an organization grows, so does its data. Choosing a databank solution that can scale along with business needs is vital.
- Integration Capability: The chosen solution must integrate effectively with existing systems and workflows for smooth data flow.
- Cost-Efficiency: Analyze the total cost of ownership. Itβs important for the solution to provide a balance between cost, features, and performance.
- User Experience: A user-friendly interface aids in faster adoption by staff members, which is essential for maximizing the solutionβs utility.
End
The conclusion of this article serves as a critical synthesis of the various elements that have been explored regarding databanks. Its importance lies in its ability to encapsulate the insights gained from each section, emphasizing not only what databanks are but how they play a fundamental role in various industries today.
Summarizing Key Insights
In summary, databanks facilitate efficient data storage, management, and retrieval. They are classified into various categories, including public, private, and project-specific databanks, each serving distinct purposes. The technological framework underpinning these systems, which include database management systems and cloud-based solutions, further enhances their utility.
Business intelligence relies heavily on databanks for data-driven decision-making and performance metrics. The applications of databanks are extensive, impacting sectors like healthcare, finance, and marketing. However, concerns surrounding ethics, data governance, and security vulnerabilities are significant and warrant attention.
The future of databanks is poised for transformation with trends like artificial intelligence integration and blockchain applications, which could redefine how data is managed and processed.
"Understanding the mechanics and implications of databanks allows organizations to leverage them effectively, strengthening their operational processes and decision-making skills."
Looking Ahead
As we look to the future, it is essential to monitor ongoing technological developments that affect databanks. Innovations such as AI and blockchain promise to enhance data integrity and accessibility, influencing how businesses operate. Organizations must remain agile and prepared to adopt new strategies to onboard these technologies effectively.
Moreover, the ethical implications surrounding data usage will continue to be paramount. Businesses should prioritize compliance frameworks while also ensuring that data privacy is upheld. Adapting to these trends and challenges will determine how effectively databanks are utilized and how they continue to transform the landscape of data management.
Ultimately, databanks are not just repositories of information; they are influential tools that, when used wisely, can lead to far-sighted decision-making and improved operational efficacy.