Exploring Location Intelligence: Implications and Applications
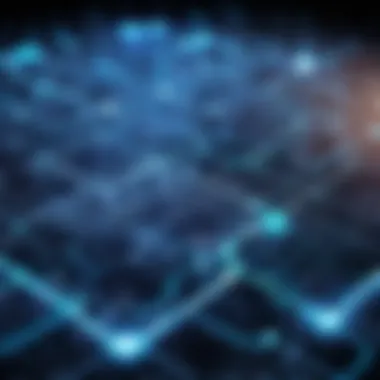
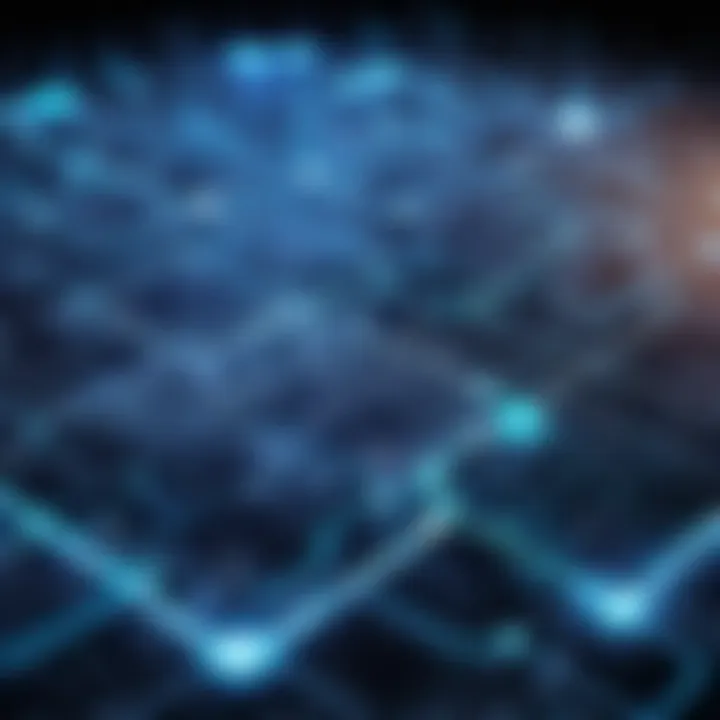
Intro
Location intelligence (LI) is becoming essential in today's data-driven world. It helps organizations understand geographical data, analyze trends, and optimize decision-making. In many industries, understanding location data aids in making strategic choices. This article will explore the core aspects of LI, focusing on its methodologies, technologies, and real-world applications.
Key Features
Overview of Features
Location intelligence employs various methods and tools designed to gather and analyze geographic data. Key features often include:
- Data Visualization: LI transforms raw geographical data into visual formats, making complex information easier to understand.
- Spatial Analysis: This involves evaluating data related to physical space, revealing patterns and trends that can inform business strategies.
- Integration Capabilities: LI can work alongside other data sources and systems, enhancing the breadth of analysis and insight available.
- Real-Time Data Processing: Access to live data significantly increases responsiveness in decision-making, providing organizations the agility to react quickly to changes.
Each of these features contributes to a more nuanced understanding of how geographic locations affect business performance and strategy.
Unique Selling Propositions
Companies adopting location intelligence benefit greatly from unique propositions that set LI apart:
- Improved Decision-Making: By utilizing spatial data, businesses can make informed choices that align with their geographical context.
- Targeted Marketing Efforts: Businesses can identify and segment customer bases more effectively, crafting marketing strategies that reach the right audience in the right location.
- Operational Efficiency: LI helps streamline operations by evaluating logistical routes and supply chains based on location data.
- Risk Management: Understanding geographical factors enables organizations to anticipate and mitigate risks associated with natural disasters, geopolitical changes, or market dynamics.
These selling points highlight the strategic advantages that can be gained through the thoughtful integration of location intelligence.
Pricing Models
Different Pricing Tiers
When considering location intelligence solutions, organizations encounter various pricing models. Many providers offer tiered subscription plans, which may include options such as:
- Basic Tier: Typically provides core functionalities such as data visualization and basic analysis tools.
- Standard Tier: Often includes additional features like advanced analytics and integration capabilities, suitable for many mid-sized companies.
- Premium Tier: Designed for large enterprises, offering the most comprehensive tools, including high-level data processing and custom support.
The choice of pricing tier can greatly affect the level of insight and analytical capabilities available to an organization.
ROI and Cost-Benefit Analysis
Investing in location intelligence software requires careful consideration of potential returns on investment. A well-executed cost-benefit analysis might include factors like:
- Increased Revenue Opportunities: Access to detailed insights can open new markets and improve existing customer relationships.
- Operational Savings: Optimized routes and supply chains typically reduce costs, enhancing overall financial performance.
- Enhanced Competitive Advantage: Organizations that leverage LI insights can gain a significant edge over competitors slow to adopt data-driven decision-making.
Thus, understanding the financial implications and potential returns is critical when assessing location intelligence solutions.
"In the age of data, location intelligence is not just an optional tool; it is a necessity for strategic growth and operational efficiency."
By understanding key features, pricing models, and potential ROI, organizations can decide how best to integrate location intelligence into their strategic endeavors.
Understanding Location Intelligence
Location Intelligence (LI) holds significant weight in todayβs data-driven landscape. It transforms geographical data into meaningful insights that organizations can leverage for strategic decision-making. As businesses and industries are increasingly embracing the integration of spatial awareness into their operations, understanding LI becomes a vital requirement.
Definition and Importance
Location Intelligence refers to the process of deriving insights from geospatial data. This data is often associated with specific locations on Earth, which can inform various business strategies. Its importance lies in its ability to enhance decision-making by providing context that traditional data may lack. Effective use of LI can lead to increased operational efficiencies, targeted resource allocation, and improved customer experiences.
Historical Context
The evolution of Location Intelligence reflects advancements in technology and data analytics. Historically, LI started from simple mapping tools to the sophisticated geographic information systems (GIS) we see today. The rise of technology such as GPS and remote sensing has only accelerated its development. Organizations now recognize the power of location data, enabling better strategy formulation and operational execution.
Key Components
Understanding the main elements that constitute Location Intelligence is essential for its effective implementation. This encompasses three key components: Geospatial Data, Data Analytics, and Visualization Tools.
Geospatial Data
Geospatial Data is crucial as it forms the foundational layer for Location Intelligence. The specific aspect of this data includes various forms such as satellite images, maps, and spatial datasets. Geospatial Data is characterized by its association with geographic locations, making it a dominant choice for analyses that require spatial dimension. Its unique feature is the ability to represent complex phenomena in tangible formats, connecting them to places on a map.
The advantages of Geospatial Data include its accuracy and relevance in analyses that span practical applications in urban planning, transportation routing, and disaster management. However, it can be subject to challenges like spatial data quality and accessibility, which may limit its effectiveness in certain analyses.
Data Analytics
Data Analytics serves as the examination of this geospatial data to extract actionable insights. Its key characteristic is the application of statistical techniques and algorithms to analyze trends and patterns within the data. Data Analytics is a beneficial choice in this context, as it allows organizations to uncover insights that direct business strategies and operational changes.
A unique feature of Data Analytics is its capability to process vast quantities of data quickly, refining it into intelligible information. Nevertheless, the complexity of the analytical models may present a challenge, requiring skilled personnel to interpret the results accurately and effectively.
Visualization Tools
Visualization Tools play a pivotal role in interpreting the data derived from Geospatial Data and Data Analytics. These tools are characterized by their ability to create visual representations of complex datasets, making the information accessible and understandable at a glance. Visualization Tools are an advantageous choice due to their ability to highlight trends, outliers, and spatial relationships, making them crucial for effective communication of findings.
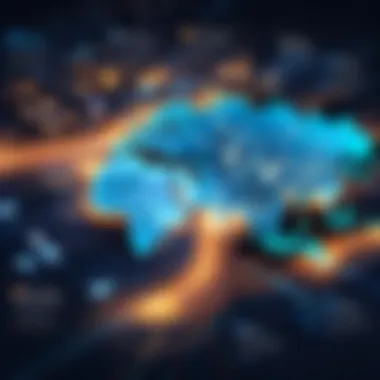
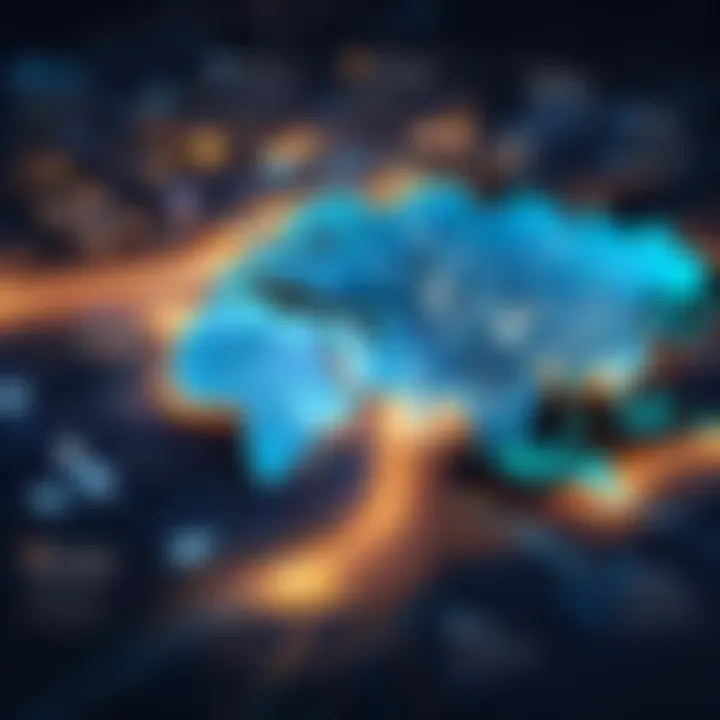
The unique feature of these tools lies in their capacity to turn data points into engaging maps and charts that foster better comprehension of underlying issues in the data. However, it is essential to recognize that poor visualization choices may lead to misinterpretations, thus emphasizing the need for thoughtful implementation.
Technological Foundations of Location Intelligence
Understanding the technological foundations of location intelligence is crucial for grasping its potential and impact across various sectors. Location intelligence leverages geographical data, enabling organizations to make informed decisions driven by spatial context. The technologies that underpin this field directly contribute to accuracy, efficiency, and the depth of insights derived from data analysis. As businesses embrace data-driven approaches, the integration of these technologies becomes essential to optimize operations and enhance strategic initiatives.
Geographic Information Systems (GIS)
Geographic Information Systems (GIS) are a cornerstone of location intelligence. GIS captures, stores, analyzes, and manages spatial data. The ability to layer different datasets allows for thorough examination and visualization of geographic trends. This capability helps organizations identify patterns and correlations that are not readily apparent in traditional data analysis. For instance, retail firms can use GIS to understand customer demographics in specific regions, aiding in location selection for new stores.
Key benefits of GIS include:
- Enhanced Analysis: It provides tools for spatial analysis that traditional data platforms lack.
- Visualization Capabilities: Users can create maps that depict complex data visually, making it easier to interpret.
- Informed Decision-Making: Organizations can make decisions based on insights derived from a geographical context, minimizing risk.
Remote Sensing Technologies
Remote sensing technologies utilize satellite or aerial imagery to gather data about the Earth's surface. This technology helps in monitoring environmental changes, urban development, and even natural disasters. Remote sensing provides valuable data that augment traditional datasets, offering insights that are timely and often more difficult to gather through conventional means.
Some uses of remote sensing include:
- Environmental Monitoring: Tracking changes in land use and vegetation.
- Urban Planning: Assessing urban sprawl and infrastructure needs.
- Disaster Management: Rapid assessment of damage following natural disasters helps in emergency response efforts.
The Role of Big Data
The concept of big data plays a pivotal role in location intelligence. The sheer volume of data generated today from various sources such as social media, IoT devices, and transactional systems enriches location datasets significantly. Analyzing this data requires significant computational power and sophisticated analytic tools.
Key considerations regarding big data include:
- Data Variety: Location intelligence can draw from diverse data sources, leading to more robust insights.
- Real-Time Analysis: Organizations can react nimbly to changing conditions, as big data allows for immediate data processing.
- Predictive Capabilities: The integration of big data analytics facilitates prediction of future trends based on historical location data.
Machine Learning Algorithms
Machine learning algorithms enhance the capabilities of location intelligence by automating data analysis and improving prediction accuracy. These algorithms can identify complex patterns in large datasets that often evade human analysis. Evolving from simple predictive models to sophisticated neural networks, machine learning is integral to advancing location intelligence methodologies.
Considerations in machine learning include:
- Scalability: As data grows, machine learning models can adapt and operate effectively on expanding datasets.
- Continuous Improvement: Algorithms can learn from new data over time, enhancing their accuracy and relevance.
- Automation: Tasks such as data cleaning and analysis can be automated, saving time and resources for organizations.
"The integration of AI and machine learning into location intelligence allows for deeper insights and significantly improved decision-making capabilities."
The interplay of GIS, remote sensing, big data, and machine learning constitute the technological bedrock of location intelligence. Through these systems and innovations, organizations can navigate complex spatial data landscapes, gaining a competitive edge in their respective industries.
Data Sources in Location Intelligence
Understanding the kinds of data sources available is fundamental to effectively utilizing location intelligence. The sources of data can significantly impact the quality and scope of insights derived from geospatial analysis. Leveraging appropriate data sources allows organizations to draw accurate conclusions and make informed strategic decisions.
Data sources in location intelligence can be classified mainly into three categories. Each category has its own attributes, benefits, and considerations that affect how data is used in different contexts.
Publicly Available Datasets
Public datasets serve as a rich resource for organizations without the budget for high-end data procurement. This data may include information from governmental agencies, such as census data, environmental data, and transportation networks. Various studies have shown that publicly available datasets can form the backbone of many location intelligence initiatives.
For instance, the United States Census Bureau offers extensive datasets that are regularly updated. These datasets provide geographical and demographic information that can inform urban planning, market research, and public services. They are also generally free to access, thus encouraging smaller organizations to adopt location intelligence tools.
However, users must be aware of potential limitations. Public datasets may not always be updated frequently, and the granularity of the data may not meet all user needs. Thus, it is crucial for organizations to evaluate the relevance and timeliness of these datasets before relying on them for critical analyses.
Commercial Data Providers
Commercial data providers bring more tailored and comprehensive datasets to the table. Firms like Esri or Pitney Bowes offer specialized datasets that focus on specific industries or types of analysis. This can be particularly beneficial for sectors like retail or healthcare, where targeted information catalyzes decision-making.
The data provided varies from consumer behavior patterns to market trends and is often enriched with analytics capabilities. Because commercial datasets come with a price tag, organizations must assess their needs and budget. Choosing an appropriate data provider hinges on the quality of data, integration capabilities with existing tools, and support offered. Each aspect plays a role in determining the effectiveness of the location intelligence strategy.
Crowdsourced Contributions
Crowdsourced data adds another layer of depth to the location intelligence pool. Platforms like OpenStreetMap allow users to contribute real-time data, driving a sense of community and collaboration. This approach has led to abundant datasets that cover geographical features, local businesses, and even traffic conditions.
One of the notable benefits of crowdsourced data is its speed and responsiveness. As communities update information almost instantly, this reflects real-world changes that traditional methods may lag behind.
However, it is important to consider the accuracy and reliability of this kind of data. Since contributions come from various individuals, the quality can vary. Therefore, establishing protocols for validating and filtering crowdsourced data is essential to ensure that it meets the necessary standards before being integrated into an organization's location intelligence toolkit.
"The effectiveness of location intelligence often hinges on the reliability and context of the data sources being utilized."
Analytical Techniques in Location Intelligence
Analytical techniques in location intelligence are crucial for extracting valuable insights from geographical data. These methods allow organizations to make informed decisions by identifying patterns, trends, and behaviors related to specific locations. As the volume of data grows, understanding these analytical techniques becomes essential for leveraging location intelligence effectively.
By employing various analytical methods, organizations can enhance their operational efficiency and effectiveness. Analytical techniques facilitate an understanding of spatial relationships, enabling companies to predict outcomes, optimize resources, and enhance customer engagement. However, the implementation of these methods must be approached with consideration of data quality and the specific context in which they are applied.
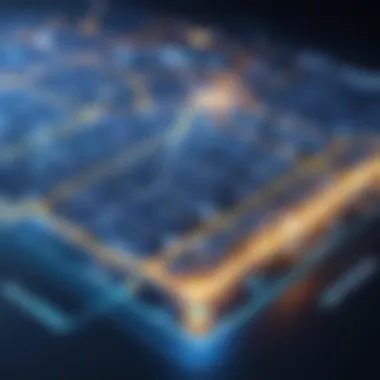
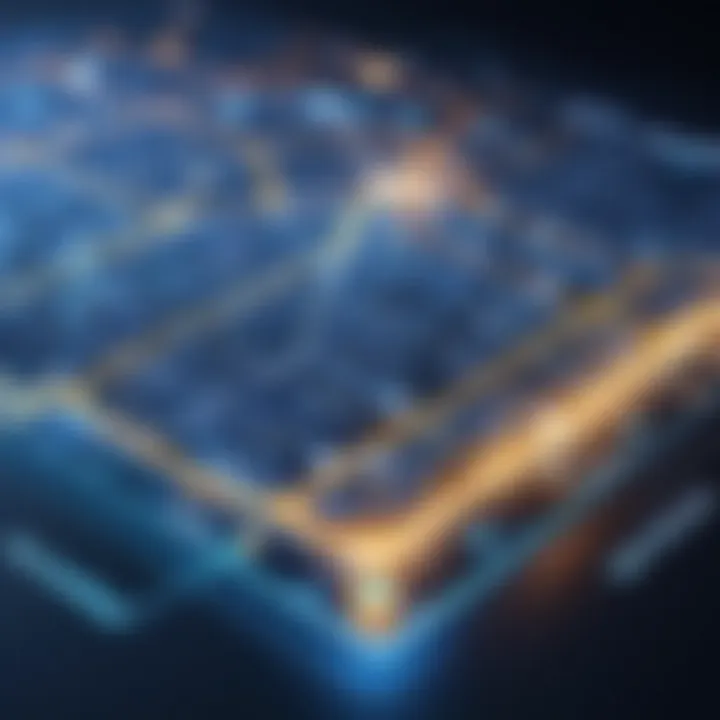
Spatial Analysis
Spatial analysis is the foundation of location intelligence. It focuses on the relationships between spatial features and the context in which they appear. This technique allows organizations to understand geographical phenomena, evaluate proximity, and analyze patterns over space. Common applications of spatial analysis include determining optimal locations for new retail stores or analyzing the geographical spread of a disease outbreak.
Key aspects of spatial analysis include:
- Geospatial Data Interpretation: Extracting meaning from data plotted on maps.
- Pattern Recognition: Identifying trends over time and space.
- Proximity Analysis: Evaluating the distance between features, which can reveal important insights for business decisions.
To conduct spatial analysis effectively, tools like Geographic Information Systems (GIS) are often utilized. These systems support the integration of spatial data with traditional databases, facilitating advanced analysis.
Predictive Modeling
Predictive modeling focuses on using statistical techniques to forecast future events based on historical data. In location intelligence, this approach can help organizations anticipate customer behavior and market trends. Through the analysis of past events and spatial data, businesses can derive models that predict future outcomes.
There are several key elements involved in predictive modeling:
- Data Collection: Gathering historical geospatial data that is relevant to the analysis.
- Model Development: Creating algorithms that capture the relationships between input variables and predicted outcomes.
- Validation: Testing the predictive model against new data to ensure accuracy and reliability.
Using predictive modeling can empower organizations to optimize decisions such as inventory management, site selection, and targeted marketing efforts based on expected consumer behavior.
Clustering Techniques
Clustering techniques segment data into distinct groups with similar attributes. This approach is significant in location intelligence as it enables organizations to identify natural groupings within their data. For instance, clustering can help identify areas with high customer concentrations or regions with similar characteristics in socio-economic factors.
Key points related to clustering techniques include:
- K-means Clustering: A popular method that partitions data into a specific number of groups based on distance metrics.
- Hierarchical Clustering: A method that builds a tree of clusters, allowing for varying levels of detail in the grouping.
- Application Areas: Clustering is widely used in market segmentation, customer profiling, and urban planning.
By employing clustering techniques, organizations can refine their strategies to better target specific demographics or service areas. This can enhance marketing campaigns or inform public policies that rely on geographical insights.
Applications of Location Intelligence Across Industries
Location Intelligence (LI) offers transformative opportunities across various sectors. The power of geospatial data combined with analytics can drastically improve decision-making processes. Various industries capitalize on LI to streamline operations, enhance customer experience, and drive strategic initiatives. As organizations increasingly rely on data-driven strategies, understanding its applications is crucial.
Retail and E-commerce
In retail, location intelligence plays a vital role in determining market strategies. By analyzing demographic data along with consumer behavior patterns, businesses can identify optimal store locations. Additionally, retailers use LI to manage supply chains effectively, ensuring products are available where demand is highest. For instance, store placement can be guided by insights derived from walking patterns and local purchasing habits.
Some advantages include:
- Improved inventory management.
- Enhanced promotions tailored to local markets.
- Targeted advertising based on the geographic distribution of customers.
With the rise of e-commerce, understanding delivery routes through location data can also optimize logistics, ensuring timely shipments and reducing costs.
Healthcare
Healthcare systems benefit immensely from location intelligence by enhancing resource allocation and patient care. By mapping disease outbreaks and tracking health trends, institutions can effectively allocate resources where they are needed the most. Furthermore, hospitals can analyze patient demographics and geographic data to improve community outreach and services.
Key applications involve:
- Identifying areas with high prevalence of specific health conditions.
- Optimizing ambulance routes to reduce response times.
- Facilitating telehealth services by understanding patient locations.
This intelligence allows healthcare professionals to act effectively during public health emergencies, ensuring timely intervention.
Transportation and Logistics
Transportation and logistics represent perhaps the most obvious applications of location intelligence. Real-time navigation and traffic data allow companies to optimize routes, saving time and fuel. Companies like Uber and FedEx leverage LI to route their services efficiently based on current conditions.
The implications include:
- Enhanced route optimization resulting in lower operational costs.
- Improved punctuality in delivery services.
- Predictive analysis to foresee traffic trends based on historical data.
Moreover, businesses can utilize location data to assess the performance of different logistics centers, leading to improved decision-making in expanding service areas.
Real Estate
In real estate, location intelligence helps investors and developers identify emerging markets. Analyzing geographical data enables stakeholders to assess property values, understand community demographics, and contemplate urban development trends. By utilizing LI, investors gain insights about what locations will yield the highest returns.
Some considerations are:
- Analyzing zoning regulations and their influence on property development.
- Assessing accessibility to amenities and transportation.
- Understanding local market fluctuations influenced by geographical factors.
Real-time data combined with predictive modeling can significantly minimize risk when making investment decisions.
Government and Public Sector
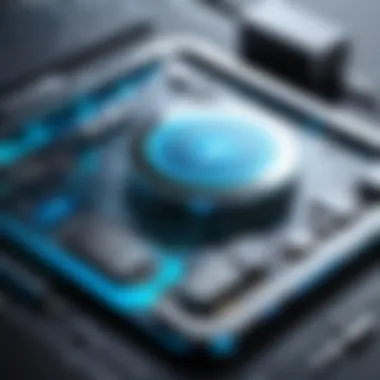
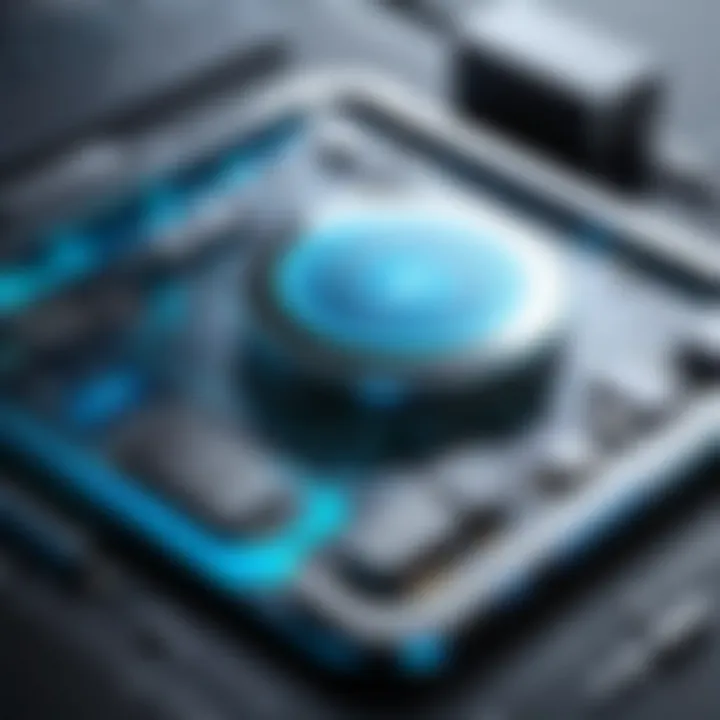
Governments harness location intelligence for urban planning, emergency management, and policy formulation. By leveraging geospatial analytics, public sector organizations can enhance service delivery and engage effectively with citizens. For instance, during emergencies, LI aids authorities in deploying resources efficiently and assessing damage while ensuring swift recovery efforts.
The benefits include:
- Better allocation of public services based on demographic insights.
- Enhanced community engagement through data-driven policy making.
- Improved resource management and efficiency in public safety responses.
In summary, the applications of location intelligence across various industries underscore its significance as a tool for strategic decision-making. Organizations adopting LI can drive not only operational efficiency but also innovate processes that connect more effectively with their customers.
Challenges and Considerations in Location Intelligence
Understanding the challenges and considerations in location intelligence is crucial for businesses embrace this valuable tool. While location intelligence offers numerous benefits, it also comes with its own set of complexities that must be navigated carefully. These challenges can impact the overall effectiveness, security, and accuracy of location-based insights. Addressing them is essential to harness the full potential of LI technologies.
Data Privacy and Security Concerns
Data privacy stands as one of the foremost challenges faced by organizations utilizing location intelligence. The gathering of geospatial data often involves capturing sensitive information about individuals, which can lead to serious privacy implications. Companies must comply with various regulations such as the General Data Protection Regulation (GDPR) in Europe. Ensuring that data is aggregated and anonymized is critical. Failure to protect this data can result in legal repercussions and damage to an organizationβs reputation.
Moreover, cyber threats can compromise the security of location data. Security breaches may lead to unauthorized access to sensitive information, exposing organizations to risks of fraud and identity theft. Investing in robust cybersecurity measures is necessary to protect location data and maintain consumer trust.
Integration with Existing Systems
Another challenge lies in integrating location intelligence solutions with existing systems. Many organizations have legacy systems that may not support advanced location data tools. This can create inefficiencies and hinder the seamless flow of information across departments. Companies must evaluate their current infrastructure and invest in necessary upgrades to ensure compatibility.
Taking a staged approach, where businesses gradually integrate new technology, can help minimize disruptions. Collaboration between IT and business units is vital to identify synergies and address integration issues proactively.
Quality and Accuracy of Data
The quality and accuracy of information used in location intelligence directly affects decision-making outcomes. Inaccurate geospatial data can lead to misguided strategies, resulting in wasted resources and missed opportunities. To ensure reliable insights, organizations must prioritize data validation processes. This may involve cross-referencing multiple data sources or employing validation algorithms to detect anomalies.
Furthermore, the currency of data is important. Outdated information can skew analysis and insights, especially in rapidly changing environments, such as urban development or healthcare. Regular updating of datasets is essential to maintain accuracy and relevance in analyses.
"Quality data is not just an asset; it is a fundamental prerequisite for actionable insights."
In summary, while location intelligence offers tremendous potential, organizations must navigate data privacy concerns, integration with existing systems, and the need for high-quality, accurate data. Recognizing and addressing these challenges will enhance the strategic value brought by location intelligence tools.
Future Trends in Location Intelligence
As we look ahead, the future of location intelligence presents many opportunities that can significantly transform how businesses operate. Understanding these trends is crucial for organizations that wish to remain competitive in an increasingly data-driven environment. Emphasis on emerging technologies, sustainable practices, and the evolving needs of consumers will shape the landscape of location intelligence in the coming years.
Artificial Intelligence Integration
The integration of artificial intelligence (AI) into location intelligence systems represents a significant leap forward. AI can process vast amounts of geospatial data more efficiently than traditional methods. This capability enables organizations to gain timely insights and make more informed decisions. A notable benefit is the ability to automate data analysis, reducing the reliance on human intervention.
AI-driven algorithms can identify patterns and trends in geospatial data that might go unnoticed. Furthermore, machine learning models can improve over time, increasing accuracy in predictive analytics. For instance, retail businesses can use AI to analyze foot traffic patterns and optimize their store locations. This not only increases revenue but also enhances customer experience by meeting them where they are likely to shop.
Advancements in Real-time Data Processing
Real-time data processing is another critical trend that is reshaping location intelligence. The ability to capture and analyze data instantly allows stakeholders to respond promptly to changing scenarios. This immediacy is particularly vital in situations such as disaster response, where timely information can save lives.
Real-time processing also enhances dynamic decision-making in logistics and supply chain management. Companies can track shipments and monitor conditions to optimize delivery routes. By leveraging IoT devices, organizations can gather geospatial data and process it in real-time, leading to enhanced operational efficiency.
Sustainable Urban Development
The emphasis on sustainable urban development is prompting a shift in how location intelligence is applied. Urban planners and governments are increasingly using location intelligence to design smarter, greener cities. Geographic insights assist in analyzing infrastructure projects, transportation networks, and even socio-economic factors affecting urban growth.
For example, location intelligence can help identify areas that would benefit from green spaces, thus improving air quality and community wellbeing. This approach to urban development can lead to reduced carbon footprints and better resource management.
"Incorporating geospatial insights into urban planning fosters sustainable growth and enhances quality of life."
The End: The Role of Location Intelligence in Strategic Decision Making
Location intelligence (LI) serves as a pivotal framework for organizations looking to enhance their decision-making capabilities through the lens of geographical context. The significance of LI is underscored by its ability to translate complex data into actionable insights. This approach not only streamlines operations but also fosters informed strategic planning and resource allocation. In essence, LI equips decision-makers with the tools they need to visualize data in relation to geographical elements, enabling them to pinpoint trends, anticipate challenges, and seize opportunities.
The benefits of integrating location intelligence into strategic decision-making are multi-faceted. Organizations can leverage geospatial data for various purposes, such as market analysis, customer segmentation, and risk evaluation. For instance, businesses can identify the best locations for new stores by evaluating foot traffic, demographics, and competitor presence. Similarly, municipal governments use LI for urban planning and infrastructure management, ensuring resources are allocated efficiently to improve public services.
However, businesses must also consider challenges that come along with the implementation of LI. Data quality and privacy remain top concerns. Organizations must prioritize accurate data collection and implement robust data governance strategies to protect sensitive information.
"The integration of location intelligence in decision-making not only enhances operational efficiency but also drives innovation within organizations."
In summary, the role of location intelligence extends beyond mere geographical analysis. It fundamentally reshapes how organizations think about their strategic initiatives, allowing them to respond effectively to their environment and make data-driven decisions that lead to long-term success.
Recap of Key Insights
In this article, several key insights have emerged that underline the importance of location intelligence in strategic decision-making:
- Enhanced Data Visualization: LI transforms raw data into geographic visualizations, making patterns and trends more understandable.
- Improved Operational Efficiency: By utilizing geospatial data, organizations can streamline processes, leading to reduced costs and optimized workflows.
- Informed Decision-Making: Decision-makers can rely on data-driven insights that connect location-based factors with business outcomes.
- Strategic Resource Allocation: Organizations can allocate resources more effectively by understanding spatial dynamics and market needs.
Implications for Future Research
Future research regarding location intelligence should focus on several areas that can enhance its applications and address the existing challenges:
- Ethics of Data Usage: How can organizations balance the advantages of LI with ethical considerations, particularly in terms of privacy?
- Integration with Artificial Intelligence: Researching how AI can further enhance the capabilities of LI tools may yield significant efficiencies.
- Advanced Predictive Analytics: Developing more sophisticated predictive analytics models that utilize LI can help organizations forecast trends more accurately.
- Real-World Application Studies: Detailed case studies across different sectors can provide a clearer understanding of practical applications and the benefits of LI implementation.
Understanding these areas not only contributes to the evolution of location intelligence but also strengthens its relevance in the changing landscape of data-driven decision-making.