Exploring Data Vault for Mac Users: A Business Perspective
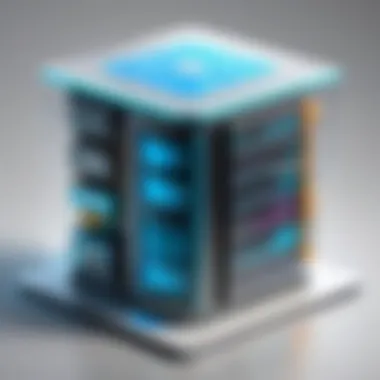
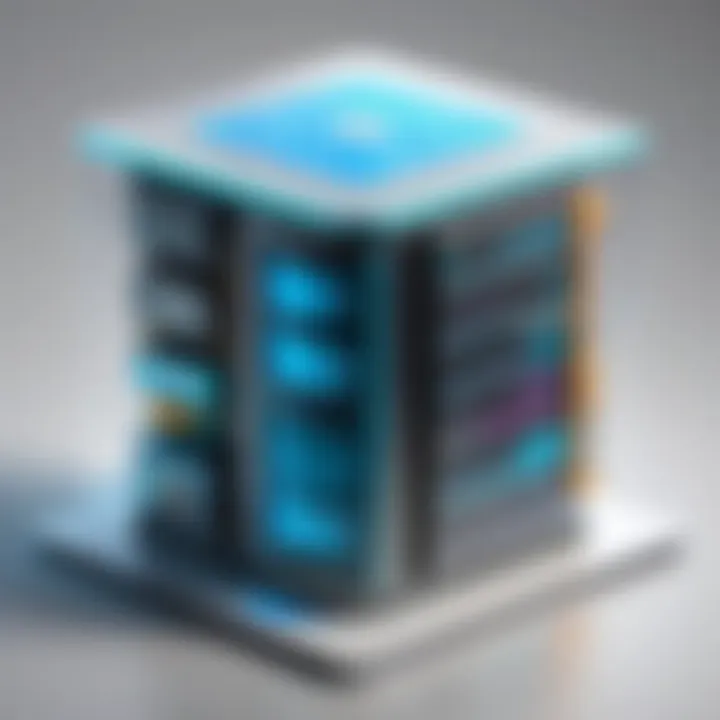
Intro
In the world of data management, keeping things under control is key. For business decision-makers, understanding how various methodologies can be implemented is essential. One such approach, the Data Vault model, presents a unique solution for organizing and analyzing data. This guide zeroes in on how Mac users can harness this methodology effectively in their B2B environments.
Todayโs business climate demands agility and efficiency. Enter Data Vault, especially tailored for Mac users. This methodology is not just a buzzword; it's a robust framework that allows companies to build a flexible data warehouse, making navigation through vast quantities of information less daunting. With the right tools, this model can help streamline both operational and strategic decisions, leading to more informed outcomes.
In this article, we will explore the fundamental aspects of Data Vault. Weโll break down features, pricing models, benefits, and the challenges involved in its integration into the Mac ecosystem. Whether you are an IT professional or a business owner, understanding Data Vaultโs nuances is imperative.
Letโs embark on this journey of discovery, equipping ourselves with knowledge that can ultimately lead to better decision-making in todayโs data-heavy business environment.
Foreword to Data Vault
In today's data-driven world, understanding how to effectively manage and utilize data is more crucial than ever for businesses. Data Vault is a methodology designed to address the complex challenges of modern data warehousing. It provides a blueprint for organizations looking to scale their data operations while maintaining high standards of quality and accessibility.
By focusing on integrating data from various sources, Data Vault allows businesses to create a unified view of their information. This not only enhances decision-making but also facilitates better analytics by ensuring that all relevant data is readily available. Business decision-makers, IT professionals, and data analysts can all benefit from the insights derived from implementing this strategy.
Definition of Data Vault
Data Vault offers more than just a framework for data storage; itโs a comprehensive approach emphasizing agility, adaptability, and data integration. At its core, Data Vault separates data into three core components: Hubs, Links, and Satellites. This separation allows for streamlined data management and enables organizations to track changes in data effectively.
- Hubs contain unique business keys, representing distinct entities or concepts.
- Links establish relationships between these entities, providing context for data usage.
- Satellites store descriptive information and historical changes, ensuring that data auditors can access prior states of the data.
For IT leaders and business owners alike, embracing a Data Vault approach means recognizing the importance of maintaining historical data without losing sight of the operational efficiencies required for real-time analytics.
Historical Context and Evolution
Understanding the evolution of Data Vault helps contextualize its value today. Originally developed in the early 2000s, the methodology was conceived by Dan Linstedt in response to traditional data warehousing pitfalls. Prior systems often struggled with scalability and adaptability, leading organizations to face difficulties maintaining their data warehousing solutions as they grew or changed.
As the business landscape became increasingly dynamic, the need for a more robust and flexible data architecture emerged. Data Vault was thus born out of the necessity to meet these evolving demands. Its historical development has continually revolved around refining techniques to enhance the integration and automation of data processes. What was once seen merely as a solution to a pressing issue has become a vital component of modern data strategy.
"Data Vault is not just a repository of information; itโs a living ecosystem that adapts to the complexities of business change."
With its roots firmly grounded in addressing contemporary business challenges, Data Vault has steadily gained traction among organizations wanting to leverage their data more effectively. As we delve deeper into this guide, we will unpack the critical principles, advantages, and practical implementations relevant to Data Vault on Mac systems.
The Importance of Data Vault in Data Warehousing
In today's business landscape, data is often regarded as the new oil. However, without the right methodology to manage this data efficiently, organizations can find themselves with a glut of information that is both overwhelming and underutilized. This is where the Data Vault methodology comes into play. It has emerged as a pivotal framework in data warehousing, particularly notable for businesses seeking to enhance their data management framework on Mac systems.
The significance of Data Vault in data warehousing is underscored by its ability to address various critical issues that businesses face. Through a structured design, it tackles the complexities associated with data integration, offering a robust solution that promotes reliability and scalability.
Core Principles of Data Vault
At the heart of Data Vault lies its core principles, which revolve around three fundamental components: hubs, links, and satellites. These components work together to create a flexible and comprehensive architecture that represents business processes accurately. Hubs are used to store unique business keys, links illustrate relationships between different data points, and satellites hold descriptive attributes tied to those keys. This organization not only facilitates easier data retrieval but also enhances clarity in how information combines across various sectors.
One of the striking features of this design is its ability to separate historical data tracking from transactional data. In essence, it allows companies to maintain a historical record without losing the context of current business processes. This capability is invaluable for analytics, as decision-makers can access timely, relevant data when making choices, leading to enhanced insight into trends and patterns.
Advantages of Data Vault Methodology
The attractiveness of the Data Vault methodology lies largely in its myriad advantages, making it a favorable choice for organizations, especially those operating within B2B environments. Here are some of its standout characteristics:
Scalability
Scalability is a pivotal aspect of Data Vault. As businesses grow and evolve, their data requirements invariably change. The architecture of Data Vault supports this expansion gracefully. Instead of requiring a complete overhaul of the system as new needs arise, Data Vault facilitates incremental additions. This characteristic is immensely beneficial for decision-makers who are looking to future-proof their data strategy.
Moreover, because Data Vault structures can easily accommodate additional data sources or new business processes, they allow for a smoother integration process, minimizing disruptions to daily operations.
Flexibility
Flexibility is yet another hallmark of the Data Vault approach. Its design allows for modifications without significant rework, ensuring that businesses can pivot quickly in response to market changes or emerging opportunities. This makes it considerably popular among organizations that need an agile data warehousing solution.
One notable feature of this flexibility is the ability to incorporate diverse data from various platforms seamlessly. Whether itโs structured data from relational databases or semi-structured data from sources like APIs, Data Vault excels in harmonizing these elements, making it an attractive prospect for tech-savvy teams.
Historical Tracking
Then thereโs the aspect of historical tracking, which is vital for organizations aiming to understand trends over time. Data Vault embraces a time-centric model, allowing businesses to keep track of changes in their data as they happen.
This unique feature ensures that decision-makers can accurately trace how data states have evolved, providing them with deeper insights into patterns and helping to replace subjective assumptions with data-driven narratives. However, one must note that comprehensive historical tracking might require additional storage and resources, so this factor needs careful consideration during planning phases.
"Implementing Data Vault is like having a well-organized attic: you can easily find what you need, even if it's from years ago."
The implementation of Data Vault, particularly within the Mac ecosystem, offers significant advantages to organizations that prioritize data management. It creates a solid foundation that accommodates growth, adapts to change, and ensures that historical context is never lost, benefiting the analytics processes and ultimately driving better business decisions.
Data Vault Architecture
Data Vault architecture is crucial to harnessing the full potential of the Data Vault methodology, especially for Mac users. This architecture brings structure to the overwhelming piles of data that modern businesses tend to produce. The key to its efficacy lies in its flexibility, allowing organizations to evolve without having to rebuild every single component from scratch. In this section, we will explore the components of Data Vault, breaking them down into manageable pieces.
Components of Data Vault
The architecture of Data Vault consists of three main components โ Hubs, Links, and Satellites. Each of these has a distinct function that contributes to a seamless integration of data, providing a clear roadmap for the organizationโs data strategy.
Hubs
Hubs are the backbone of the Data Vault architecture. They store all the unique business entities that an organization deals with. Essentially, if you can think of the data in terms of core entities, they belong here.
Hubs are characterized by their stability and uniqueness, forming the center of business objects. This stability is highly beneficial. It is as if the Hubs provide a sturdy chart that helps navigate through the sea of data without losing oneโs bearings.
One unique feature of Hubs is that they do not contain any history; they only reflect the current state of the business entities. This can be a slight drawback. In cases where historical context is necessary, it's important to look into how other components interact with the Hubs to maintain that context.
Links
Links serve a different purpose within the architecture. They define relationships between Hubs. This is vital because real-world scenarios are hardly about isolated entities; rather, itโs about how those entities intersect.
The primary characteristic of Links is their flexibility. They let organizations model relationships as they evolve, ensuring that their data architecture is agile and can adapt swiftly to different business needs.
A unique feature here, however, is that Links store the relationships only. They come without any historical data. This could present a challenge when organizations want to visualize their evolving relationships over time. The context is everything, and organizations might need strategies to bridge that gap when needed.
Satellites
Satellites carry the load of historical data for Hubs and Links. They track changes and provide a way to see how entities have evolved over time.
What makes Satellites particularly useful is their ability to deliver a rich history, which can aid decision-making processes. Think of Satellites as the diaries of both the Hubs and Links. They recount events and changes, making it easier for analysts to understand trends and movements within the business.
One downside to Satellites is that they can significantly increase storage requirements. For businesses operating in environments where data space is at a premium, carefully managing these storages becomes crucial.
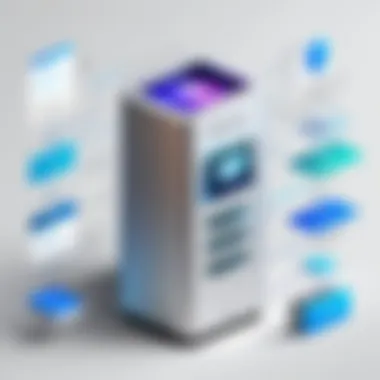
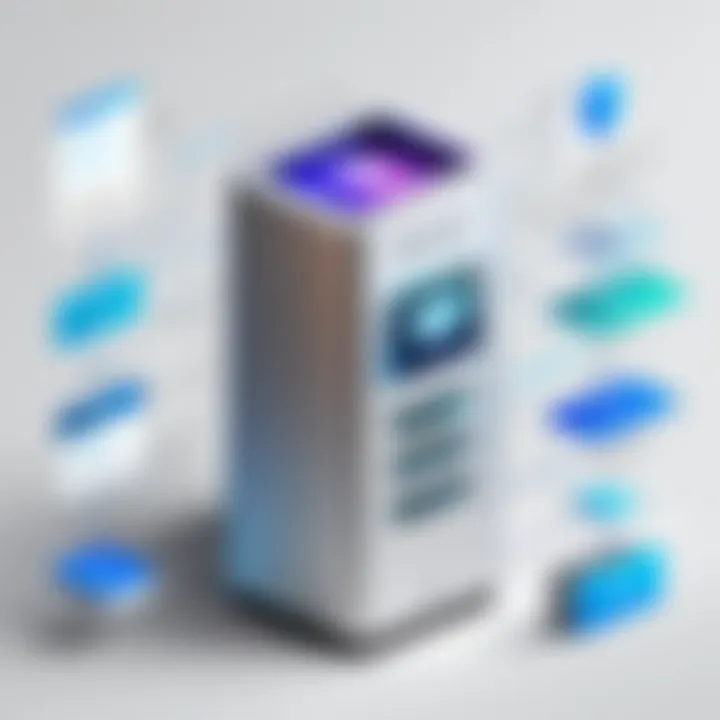
Data Vault Modeling Techniques
Understanding the components is only part of the equation; itโs just as crucial to use the right modeling techniques to make the most of those components. Data Vault modeling emphasizes simplicity, flexibility, and scalability โ three key tenets that empower businesses to adapt to changing data landscapes.
The modeling process should focus on defining business keys and establishing relationships effectively. This ensures that all components align with business objectives and can support ongoing analytics efforts effectively.
System Requirements for Data Vault on Mac
When gearing up to implement Data Vault methodologies on a Mac, understanding the system requirements is essential. This aspect lays the groundwork for a smooth and successful deployment. As Data Vault is a robust method of data modeling, it demands a certain level of hardware and software support, which can affect everything from performance to ease of use.
Hardware Specifications
The hardware specifications for Data Vault on Mac revolve around ensuring efficiency and a seamless user experience. Here are some notable points to consider:
- Processor: A multi-core processor, preferably from Intel, is vital. The more cores, the better the database can handle concurrent processes without lag. A quad-core setup is generally a good baseline.
- Memory (RAM): While Macs can run Data Vault with as little as 8GB of RAM, 16GB or more is highly recommended for better performance, especially if you're dealing with large datasets. This extra memory helps in preventing slowdowns during data processing.
- Storage: SSDs are preferable over traditional HDDs due to their speed. Minimum storage should be 256GB, but if you're planning extensive data storage, consider a larger capacity or external storage solutions to avoid bottlenecks.
- Graphics: Though not often emphasized, if you're using visualization tools that work alongside your Data Vault, having a robust graphical processing unit (GPU) can improve the rendering of complex data visualizations.
Above all, the overall architecture of your Mac system should be aligned with the scale of your operations. Adequate hardware ensures that users can retrieve and manipulate data swiftly, leading to efficient decision-making.
Software Dependencies and Tools
Next, let's turn our attention to the software side of things. Data Vault on Mac isn't standalone; it relies heavily on various dependencies and tools. Here are some important aspects to keep in mind:
- Database Management System (DBMS): You should consider options that are compatible with Mac OS such as PostgreSQL or MySQL. These databases support structured data, and they can efficiently handle the insert-heavy nature of Data Vault.
- ETL Tools: For data extraction, transformation, and loading processes, tools like Talend, Apache Nifi, or Pentaho can be extremely helpful. These tools provide user-friendly interfaces and robust features to streamline your data handling tasks.
- Programming Languages: Familiarity with SQL is critical, given its central role in querying databases. Additionally, languages such as Python or R can enhance data manipulation and analysis capabilities.
- Visualization Tools: If youโre keen on interpreting data, using visualization tools like Tableau or Looker can give you insights that raw data might not immediately show. Ensure these tools integrate well with your chosen database platform.
Itโs imperative to keep your software stack updated. Compatibility issues can lead to unnecessary headaches, undermining the potential of the Data Vault framework.
A tailored setupโconnecting the right hardware and softwareโacts like a sturdy bridge, allowing you to traverse the complex landscape of data management seamlessly.
Setting Up Data Vault on Mac
Setting up Data Vault on a Mac isn't just a mere technical task; it lays the groundwork for effective data management and analytics. This process is essential for business leaders, IT specialists, and technical analysts. With the rise of data-centric decision-making, understanding how to adeptly implement this methodology on Mac systems is pivotal. It ensures seamless integration with existing workflows, thus maximizing the overall benefits of Data Vault's structured approach to data management.
The proper setup allows organizations to safeguard their data while also enhancing its accessibility. A correctly configured Data Vault can lead to significant improvements in data analysis and reporting capabilities. Additionally, with the unique environment of MacOS, there are specific considerations that users should keep in mind.
Installation Procedures
Installing Data Vault on a Mac involves several systematic steps. To start, identifying and acquiring the right tools is crucial. Unlike traditional data warehousing methods, Data Vault requires a paradigm shift. Here are the general steps to follow:
- Select the Appropriate Software: Choose a data modeling tool compatible with Mac. Some popular options include dbt, Talend, or Matillion. Each of these has its strengths and will integrate differently within the Mac ecosystem.
- Download and Install: Follow the specific installation instructions for your chosen tool. Typically, this would involve downloading the software from its official website and following the set-up wizard. Make sure system permissions are granted to avoid installation hiccups.
- Database Setup: Youโll need a database to store your Data Vault. Consider options like PostgreSQL or MySQL, both of which are Mac-friendly. Configure your database to ensure it aligns perfectly with your data model needs.
The installation phase doesnโt just end at plugging in some numbers. Take the time to explore the toolโs interface after setup, which sets the stage for subsequent configuration.
Configuration Best Practices
Once the installation is complete, the next crucial phase is configuration. This step can make or break the success of deploying Data Vault on Mac. Here are several best practices to follow:
- Define Your Business Keys: This ensures unique identification within the data model. Each hub in your Data Vault will represent a critical business entity, and establishing business keys from the outset is essential.
- Establish Relationships Early: By identifying and laying out connections between hubs and links in advance, you minimize later rework or confusion. Doing so provides clarity during data loading processes.
- Regular Backups: Always implement a backup strategy. Data loss can disrupt operations and slow down development. Regular snapshots of the model help in safeguarding against unexpected issues.
- Performance Monitoring: Configure monitoring tools to analyze query performance early and often. This is key to maintaining an efficient Data Vault on Mac. Itโs advisable to identify bottlenecks before they escalate into bigger issues.
- Documentation: Keep meticulous records of your configuration and any changes made during the setup. This documentation will prove invaluable when troubleshooting or scaling your design.
The attention to detail in the setup and configuration of Data Vault can significantly enhance its return on investment, allowing businesses to extract valuable insights from their data more quickly and efficiently.
Data Modeling Strategies Within Data Vault
Data modeling in the context of Data Vault is a crucial component for any successful implementation, especially on Mac systems. Most decision-makers recognize that how data is structured can directly influence the effectiveness of data analytics and reporting. This methodology prioritizes agility and scalability, which is particularly beneficial for businesses operating in fast-paced environments. Therefore, understanding data modeling strategies not only provides insights into the archival processes but also supports strategic decision-making in the long run.
Defining Business Keys
Defining business keys is essentially about identifying unique entities that distinguish one piece of data from another within the data warehouse. Each business key serves as the anchor for a hub in the Data Vault model. These keys are foundational because they ensure that no data is lost or misidentified during the process of loading.
When drafting business keys, itโs important to consider how these identifiers will relate to transactions over time. For instance, when dealing with a customer database, customer ID could serve as a business key. However, the model should also be flexible enough to accommodate changes in data structure without compromising integrity.
- Clear Identification: Ensure that business keys are drawn from trusted source systems.
- Consistency: Maintain consistency in how keys are defined and applied across all transactions.
- Flexibility: Design the keys with potential future expansion in mind.
Using business keys can streamline querying and enhance performance when accessing records. The better defined the keys are, the simpler it becomes to track relationships across various data points, which brings us to the next strategy.
Establishing Relationships
Once business keys are firmly established, the next step is to outline relationships among various data points effectively. Relationships in a Data Vault model are typically represented through links, which connect different hubs based on logical connections. For example, a link could tie a customer hub to an order hub, demonstrating which customers made which purchases and when.
Getting this step right is vital as it underpins the analytical capabilities of the data warehouse. Here are some considerations for establishing relationships:
- Type of Relationship: Determine whether the relationship is one-to-one, one-to-many, or many-to-many, as this will affect model complexity.
- Temporal Aspect: Understand how these relationships evolve over time. Itโs not just about capturing the current state but also historic changes that may influence future analysis.
- Documentation: Keep thorough documentation about relationships to make it easier for teams later on. Clear documentation can act as a guide for future modifications.
Important Note: The structure of relationships should allow for efficient querying since, in a business context, time is of the essence. An uncluttered relational model facilitates quicker insights into business performance, directly affecting operational decisions.
By focusing on well-defined business keys and solid relationships, organizations can leverage the power of Data Vault modeling to enhance their data management processes, enabling informed decision-making and strategic foresight.
ETL Processes in Data Vault
When discussing Data Vault, the importance of efficient ETL (Extract, Transform, Load) processes cannot be overstated. In a nutshell, these processes are vital for moving data from various sources into a centralized data warehouse. This is crucial for any business aiming to harness the power of its data to make informed decisions. Hereโs a closer look at both extraction and transformation aspects.
Extracting Data Efficiently
The initial step in the ETL workflow is data extraction. Efficient extraction involves gathering data from numerous sourcesโdatabases, APIs, files, etc.โand consolidating it for further processing. This step ensures that all pertinent information is available for analysis. For Mac users, tools such as Talend, Apache Nifi, and Fivetran can streamline this process, allowing for quick integration of disparate data streams.
One key element here is the consideration of data quality. Itโs essential to ensure that the data being extracted is clean and relevant. Dirty data can lead to misleading insights and, consequently, poor business decisions. Instead of just relying on automated extraction, incorporating manual validation can sometimes save more headaches.
"Good extraction is the foundation of smart analytics. If you start with garbage data, youโll end with garbage insights."
Considerations for Extraction:
- Source Diversity: Understand the origins of your data; whether it's from operational systems or external providers, each source has its own structure.
- Frequency of Extraction: Depending on the business needs, determining whether data needs to be extracted in real-time or batch will impact tool selection.
- Automation Capabilities: Look for tools that offer built-in automation features, which help in reducing manual effort.
Transforming Data for Storage
Once data is extracted, itโs time for transformation. This phase is where the raw data is shaped to fit the needs of the data warehouse modelโessentially preparing it for further analysis. Transformation often includes cleansing data, creating derived values, applying business logic, and ensuring that the data conforms to a standard format.
For Mac users, applications like Alteryx and Informatica could serve as useful counterparts for this transformation task. They not only offer rich features for handling complex transformations but are also user-friendly, which should appeal to business decision-makers who may not have a deep technical background.
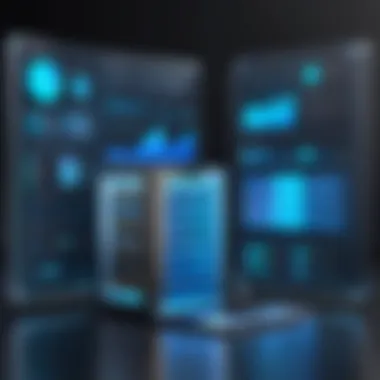
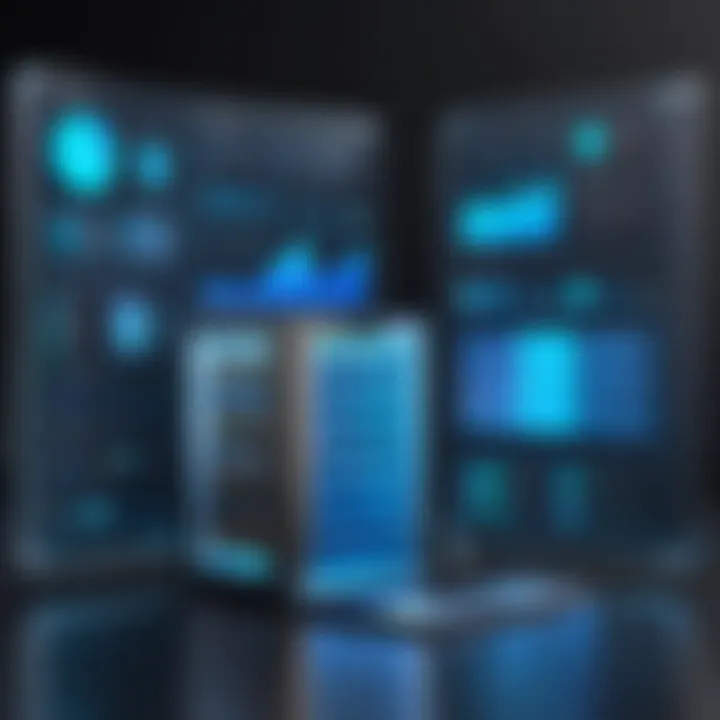
Some critical transformation processes include:
- Data Cleansing: Removing duplicates and correcting errors to maintain data integrity.
- Data Aggregation: Summarizing data points into meaningful metrics for analysis.
- Data Enrichment: Adding context or additional information to data points to enhance understanding.
- Implementing Business Rules: Establishing a framework that matches data presentation to organizational needs.
Transforming data properly ensures that the information isnโt just usable, but is also insightful and actionable. After all, just like a chef wonโt serve raw ingredients, a business shouldnโt rely on unprocessed data for decision-making.
Data Governance and Compliance
Data governance and compliance form a cornerstone in any data management framework, particularly when implementing Data Vault methodologies on the Mac platform. This segment underscores the vital role these elements play not just in adhering to regulations but also in enhancing data integrity and usability. Businesses today are navigating a multilayered landscape where data isnโt merely a byproduct of operations; itโs a strategic asset. Ensuring proper governance and compliance can significantly affect decision-making, risk management, and operational efficiency.
Ensuring Data Quality
Data quality is the bedrock of robust data governance. For organizations working with Data Vault on Mac, maintaining high data quality involves a combination of practices and evaluation criteria. Here's how some aspects interweave:
- Accuracy: Data must reflect reality. Even one incorrect entry can skew insights, frighteningly derailing business strategies.
- Consistency: Uniformity in how data is gathered and stored is essential. If one department pulls data from various sources with differing formats or definitions, confusion mounts quickly.
- Completeness: Missing data points can lead analysts down a dark alley where decisions are made without a clear view of the terrain. Ensure data sets are complete and filled with relevant information.
- Timeliness: Old data isnโt just stale; it can be misleading. Fresh, up-to-date information truly underpins effective data governance.
Incorporating automated tools can assist in monitoring and maintaining these quality metrics. For instance, a simple dashboard can alert users when data quality dips below predefined thresholds. This proactive approach helps keep data defects at bay.
Compliance Challenges and Solutions
Compliance presents its own set of hurdles, intertwining legal considerations and operational methodologies. The Data Vault models often involve intricate data flows that necessitate rigorous scrutiny regarding compliance. Some frequent issues include:
- Regulatory Understanding: It can be a challenge to stay updated on relevant regulations, especially in a global operating environment. Financial reporting standards may differ markedly across regions, requiring businesses to adapt their data practices accordingly.
- Data Security: Protecting sensitive information is not just good practice; itโs a legal mandate in many cases. When working with data that is subject to privacy laws like GDPR or HIPAA, organizations need robust mechanisms to ensure protection.
- Audit Trails: Establishing a comprehensive record of data origins and transformations can be tedious. However, such traceability is essential for demonstrating compliance during audits.
To mitigate these challenges, businesses can employ the following strategies:
- Regular Training Workshops: Educating employees on compliance requirements can be invaluable. Knowledgeable staff can ensure actions align with policies, reducing potential exposure to legal repercussions.
- Invest in Compliance Software: Utilizing tools tailored for regulatory compliance can streamline the tracking and reporting processes. This integration not only simplifies maintaining compliance but also enhances overall data governance practices.
- Create a Cross-Functional Compliance Team: By forming a team with members from IT, legal, and operational backgrounds, organizations can foster a more comprehensive approach to compliance issues, facilitating quicker resolutions and innovative solutions.
"Effective data governance and compliance arenโt just about obeying the law; they are about earning trust and building a culture that values data as an asset."
Navigating the complexities of data governance and compliance is not a trivial pursuit; it involves constant vigilance and strategic foresight. When those in charge are well equipped and informed, the entire organization benefits from a healthier data ecosystem.
Challenges of Implementing Data Vault on Mac
Implementing Data Vault on a Mac system comes with its share of hurdles. As appealing as the Data Vault methodology may sound, the reality is that translating that theoretical concept into practical application demands careful consideration of several challenges. These challenges are not just minor bumps in the road; they can have serious implications for the effectiveness and efficiency of data strategy in a business. When tackling the Data Vault methodology in the Mac environment, organizations and practitioners need to be aware of these obstacles, which could impede progress and detract from potential benefits.
Learning Curve for Users
One of the most significant challenges is the learning curve associated with Data Vault methodologies. This can be especially pronounced for teams who are more accustomed to traditional data modeling techniques or those who may not have a strong background in data warehousing. Adopting Data Vault means staff need to shift their mindset; they must grasp concepts like business keys, the difference between hubs, links, and satellites, and the intricacies of the ETL processes suitable for this framework.
- Understanding New Concepts: Unlike conventional data modeling, Data Vault introduces new terminologies and structures. Without proper training, users can find themselves bewildered, which can lead to errors in implementation.
- Training Costs: Organizations might need to invest in training programs. While some online resources exist, structured learning experiences often provide a more robust understanding.
- Time Constraints: All that training doesnโt happen overnight. For companies eager to capitalize on data insights quickly, the time needed to ramp up on a new methodology can be a sticking point.
The knowledge gap can hinder the implementation process significantly. When users lack confidence in their understanding, hesitation can creep in, leading to a sluggish adoption rate.
Integration with Existing Systems
Another major complication involves integrating the Data Vault framework with existing systems. Businesses may already have legacy systems or separate databases that are not readily compatible with the Data Vault architecture. This incompatibility leads to several challenges:
- Data Migration: Moving data from a traditional structure to a Data Vault model requires careful planning and execution. If not done correctly, there's a risk of data loss or redundancy.
- System Compatibility: Various tools and databases might not play nicely with each other. For instance, if a company uses a legacy data management system that wasnโt built with Data Vault principles in mind, significant adjustments may be necessary.
- Maintenance Efforts: Keeping different systems in sync can become a hassle. The more complex the ecosystem is, the higher the chances for discrepancies, which can complicate reporting and analytics.
In light of these challenges, a strategic approach to planning and execution becomes essential. Being proactive in addressing learning gaps and integration hurdles can certainly lead to a smoother transition into the Data Vault methodology.
"A smooth sea never made a skilled sailor."
This adage rings true in the context of Data Vault implementation, where navigating challenges is part of the journey to unlocked data potential.
Performance Optimization Techniques
In the realm of data management, performance optimization is akin to giving a well-oiled machine a fresh coat of paint. The importance of optimizing techniques is paramount, particularly when dealing with extensive datasets and complex queries within Data Vault on Mac systems. A well-optimized performance not only enhances user experience but also increases efficiency in processing and retrieving data. In the context of business decision-making, this means faster insights and more reliable data-driven strategies.
Improving Query Efficiency
When it comes to enhancing query efficiency, understanding how data is stored and accessed in Data Vault is essential. Queries are the lifeline for businesses, as they extract vital information from the warehouse. One efficient way to tackle this is through properly constructed SQL queries. Writing optimized SQL queries can significantly reduce processing time and resource usage.
A few strategies to consider include:
- Using appropriate JOINs: Instead of unnecessary complex joins, let's lean towards simple and necessary joins to keep things straightforward.
- Indexing: Creating indexes on essential columns allows the Mac system to find data quicker, speeding up queries dramatically, especially in large datasets.
- Limiting data retrieval: Instead of pulling all information, focus on the need-to-know data. Using filters will not just improve speed but also reduce memory usage.
- Analyzing query execution plans: Staying aware of how a query is executed can spotlight potential bottlenecks and offer ideas for refinements.
"Efficiency isnโt just about success; itโs about maintaining the clarity of operation amidst complexity."
Utilizing Caching Mechanisms
Caching mechanisms serve as a clever way to enhance performance. Think of caching as creating a shortcut for frequently accessed dataโlike having your favorite snack close to your workspace. In the context of Data Vault, caching can dramatically reduce the time it takes to retrieve data that users query often.
Two primary types of caching to consider are:
- Result caching: Store the results of prior queries so that repeat requests pull from this stored data rather than executing the same query again. This makes retrieving frequently accessed information much faster.
- Metadata caching: This involves keeping metadata about the data models and structures in memory. Knowing the structure quickly enables users to devise their queries without delay.
Implementing these techniques requires an understanding of the tools available on the Mac. There are several databases and frameworks that support caching natively. Having a clear strategy regarding when and how to use caching is crucial. Mismanaged caching can lead to outdated data being presented, leading to misinformed decisions. So, keeping cached data updated is equally important to deliver correct and timely insights.
Evaluating Data Vault Tools for Mac
In the landscape of data management, tools amplify the capabilities of methodologies like Data Vault. When you think about Data Vault tools for Mac, itโs not just about functionality; it's the power to enhance decision-making and streamline data workflows. Hence, selecting the right tool is crucial for decision-makers aiming to integrate Data Vault into their operations seamlessly. Key points revolving around evaluation include compatibility, key features, support, and overall efficiencyโall of which play a significant role in determining the success of your Data Vault implementation.
Feature Comparison of Available Tools
When assessing tools for Data Vault, a side-by-side feature comparison serves as a powerful decision-making aid. First off, it's essential to identify the features that align closely with your business needs. Here are notable features typically assessed:
- Data Modeling Capabilities: Some platforms offer more intuitive modeling interfaces that can simplify the design of hubs, links, and satellites. Look for tools with drag-and-drop functionality for ease of use.
- Integration Support: Ensure the tool harmonizes well with your existing systems. Whether it needs to integrate with SQL databases or perform API calls, compatibility is key.
- Scalability: As your data grows, so should your toolโs capabilities. Opt for solutions that can accommodate higher data volumes without compromising performance.
- Visualization Features: A visual representation of data flows can greatly help in understanding complex relationships. Look for tools that present dashboards or visual modeling tools as part of their offering.
- User Support and Community: Strong vendor support and an active user community can greatly influence your experience. Itโs beneficial to have access to tutorials and forums where users share insights and solutions.
Evaluating these features helps in making a well-rounded decision about which software fits your project goals.
Cost-Benefit Analysis
After understanding the features, a cost-benefit analysis is the next logical step. Itโs not just about the price tag attached to the tools; it's about the overall investment in terms of both time and resources.
- License Costs: Some tools might require a hefty licensing fee, while others may offer flexible pricing based on usage. Itโs vital to weigh the ongoing costs against the potential return on investment.
- Training and Learning Curve: Consider how much time and money will be spent training staff to use the new tool effectively. A steep learning curve can lead to increased costs in terms of lost productivity.
- Long-term Savings: A tool that saves time and reduces errors can translate into significant savings over the long term. Itโs about predictive analysis: calculating how much more efficient your operations could become can help justify costs.
- Performance Metrics: Evaluate what performance gains you expect from the tool. Do they match your organizational goals? Metrics such as speed of data retrieval and analysis turnaround time are essential to consider.
Assessing both costs and benefits requires careful consideration, but it provides a robust framework for decision-making. In the end, the goal is to align the tool's capabilities with your strategic objectives while ensuring fiscal responsibility.
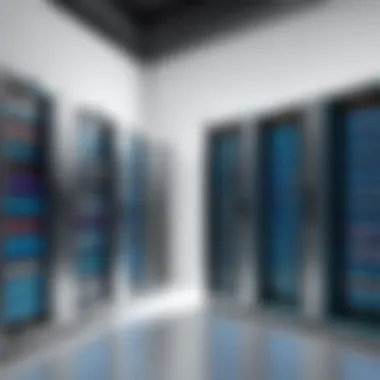
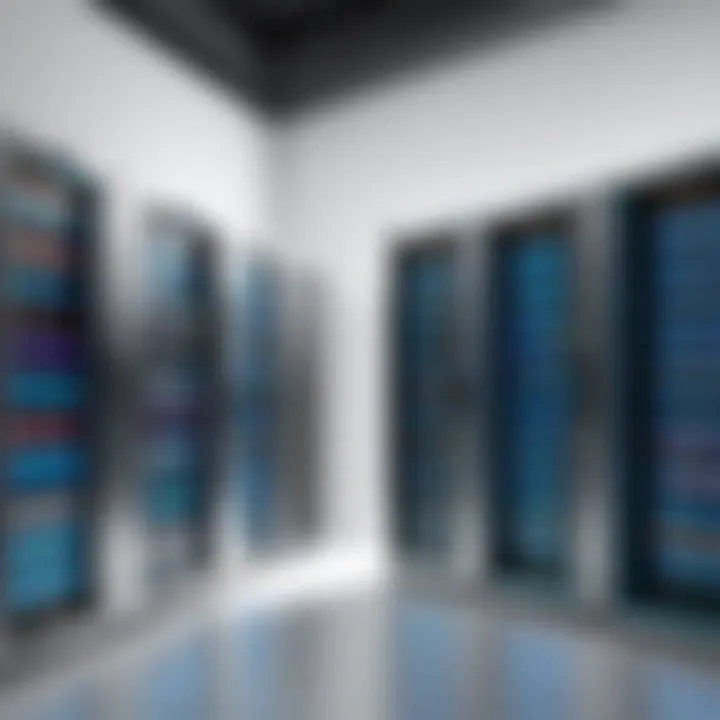
"The right tool is the one that not only fits your current needs but also has the flexibility to grow with your business."
By thoroughly evaluating tools, business leaders can make informed choices that pave the way for a successful Data Vault deployment.
Case Studies of Data Vault Implementation on Mac
The implementation of Data Vault methodologies gives decision-makers a practical lens to examine real-world applications of data strategies on Mac systems. Case studies illustrate the tangible benefits and challenges faced by organizations that have undertaken this journey. They are not just stories; they are valuable resources, demonstrating the strategic advantages of Data Vault in enhancing data management, analytics, and business agility. By delving into these examples, IT professionals and business owners can gather insights into what works, what doesn't, and how best to move forward with their data initiatives.
Success Stories
Organizations that adopted the Data Vault methodology often reap notable rewards. For instance, a prominent e-commerce company experienced significant improvements in their data handling post-implementation. Before embracing Data Vault, their data integration processes were rigid and slow, resulting in poor decision-making capabilities. By moving to a Data Vault model, they not only improved their historical data tracking but also enhanced their capacity for real-time analytics. The ability to link diverse data sources while ensuring data integrity became a game changer for them.
Another example comes from a major telecommunications provider. With each new service offering, the mountain of data grew steeper. The transition to Data Vault allowed for more streamlined data capture, leading to quicker insights into customer behavior. Now, their teams can derive actionable insights much faster, directly impacting their marketing strategies and customer service initiatives.
Such success stories showcase the versatility and effectiveness of the methodology in various industries, proving Data Vault's ability to transform data management practices on Mac systems.
Lessons Learned
While successes abound in the realm of Data Vault implementation, lessons from these ventures are equally important. One key takeaway is the significance of stakeholder engagement from the get-go. In several cases, organizations that diverted attention from buy-in among end-users during the initial phases faced resistance later on. Proper training and buy-in are not mere formalities; they are essential steps that pave the way for full adoption.
Moreover, the necessity of a solid governance framework cannot be overstated. Organizations that tended to overlook data governance encountered difficulties with data quality and compliance, which ultimately hindered their operational efficiency. Consistent methods for maintaining data integrity and tracking lineage became paramount to avoid pitfalls post-implementation.
Finally, integrating existing systems with Data Vault needs to be approached with caution. Many companies found that their legacy systems, while functional, posed certain challenges during the integration phase. Planning a phased approach with proper assessment tools can help ease transitions and minimize disruptions to ongoing operations.
Effective adoption of Data Vault is not just about technology; itโs about fostering a culture of data-driven decision-making.
Future Trends in Data Vault for Mac
The world of data management is constantly evolving, and keeping an eye on future trends is crucial, especially for businesses looking to optimize their data strategies. As more organizations pivot towards data-driven decision making, the Data Vault methodology has gained significant traction. But what does the future hold for Data Vault on Mac systems specifically? This section seeks to explore emerging technologies and predicted developments that could reshape how businesses leverage Data Vault to meet their analytics and data management needs.
Emerging Technologies
The term "emerging technologies" isn't just a buzzword; it encapsulates various innovations that have the potential to enhance the effectiveness of Data Vault implementations on Mac. Here are some of the key players in this arena:
- Artificial Intelligence and Machine Learning: The integration of AI and ML is redefining how companies handle anonymoous data within their vault. For instance, predictive analytics can help organizations forecast trends by uncovering patterns within their data silos. This sort of insight can be invaluable for making proactive business decisions.
- Cloud Computing: As businesses move operations to the cloud, the coupling of Data Vault methodologies with cloud resources is increasingly attractive. The flexibility and scalability of cloud platforms make them ideal for handling large data sets, allowing companies to implement Data Vault without the hefty infrastructure costs.
- Real-Time Data Processing: In todayโs fast-paced environment, the ability to process data in real-time is a must. New tools are emerging that can quickly ingest data and feed it into a Data Vault, thereby providing timely insights that can guide immediate business actions.
- Blockchain Technology: Though primarily known for its applications in cryptocurrency, blockchain can enhance data governance within Data Vault. With its decentralized and immutable nature, blockchain can help ensure data integrity and security, which are indispensable for any data management system.
"Staying ahead of the curve requires a willingness to adapt to new technologies. Integrating emerging tech can be a game changer for Data Vault on Mac."
Predicted Developments
As we look ahead, there are several anticipated developments that could significantly influence the landscape of Data Vault for Mac. Understanding these shifts can aid decision-makers in strategizing effectively:
- More User-Friendly Interfaces: As user experience becomes a focal point in software development, future Data Vault tools will likely embrace intuitive design principles. Simplified interfaces can reduce the learning curve for new users, especially critical for small businesses entering the data management realm.
- Standardization of Tools: Right now, thereโs a wide array of tools available for implementing Data Vault, which can be overwhelming. As the market matures, we can expect an increase in standardized platforms that focus on seamless integration and interoperability. This streamlining could help businesses allocate resources more effectively.
- Integration with Big Data Technologies: The scope of data that businesses deal with is growing at an exponential rate. Future versions of Data Vault will likely develop stronger connections with big data technologies like Hadoop and Apache Spark. Such integrations can enhance data handling capabilities, allowing users to work efficiently with vast amounts of information.
- Focus on Data Privacy: With tighter regulations around data protection, such as GDPR, future developments will likely emphasize tools that can bolster compliance. Enhancements in audit trails and regulatory reporting within Data Vault can help businesses mitigate legal risks.
In summary, the future of Data Vault for Mac appears promising, coloring a picture of adaptability and innovation. By staying proactive regarding emerging technologies and predicted developments, businesses can better position themselves to harness the full potential of their data.
Closure
In wrapping up the exploration of Data Vault methodologies within the Mac ecosystem, it becomes clear how pivotal these practices are for modern business strategies. The conclusion distills the significance of employing Data Vault for effective data management in B2B environments. Understanding this can lead to better decision-making, allowing organizations to capitalize on data analytics benefits more gracefully.
Summary of Key Takeaways
- Scalability and Flexibility: Data Vault offers a structure that can grow alongside a business. As needs evolve, adding new data sources becomes less cumbersome. This adaptability allows organizations to pivot without the usual growing pains associated with data integration.
- Historical Data Management: The ability to track historical changes seamlessly positions Data Vault as an optimal solution for businesses that require a comprehensive view of their data over time. This feature is crucial for audits and strategic planning.
- Enhanced Decision-Making: With rich data histories and flexible architectures, decision-makers are equipped to make informed choices that are backed by real data rather than gut feelings. This shift in approach can drive significant business advancements.
Final Thoughts on Data Vault for Mac
The journey through Data Vault's application on Mac systems showcases its vital role in consolidating business intelligence capabilities. By leveraging its strengths, organizations can unleash the full potential of their data assets. As emerging technologies continue to reshape the landscape, embracing solutions like Data Vault for Mac will not only simplify processes but also enhance data reliability and accuracy.
The upcoming trends in data management highlight that those who understand and implement these methodologies will likely lead the charge towards more data-driven decision-making. The landscape may change, but the strategic importance of aligning business goals with sophisticated data management Technologies will always remain at the forefront.
"In the data-driven world, the quality and management of information are as critical as the information itself."
Sara Ibrahim's insights illuminate the conversation about integrating data strategies into everyday business operations. Through an informed approach to Data Vault on Mac, businesses stand to achieve a competitive edge and elevate their data game significantly.
References and Further Reading
Understanding the subject matter of Data Vault methodologies is pivotal for anyone delving into data management and analytics, particularly for Mac users. The section on references and further reading serves not just as a collection of sources but as a critical resource for deepening knowledge and enhancing practical application. It helps the reader navigate through the complexities of Data Vault concepts, offering insights that can significantly influence business decision-making.
Diving into this pool of knowledge allows decision-makers and IT professionals to familiarize themselves with tried-and-true literature, methodologies, and case studies. Here they can grasp the foundational principles, advantages, and potential pitfalls. Having reliable references emphasizes credibility and can guide new adopters toward optimal practices for implementing Data Vault in a Mac environment.
Also, a well-curated reading list presents multiple perspectives. From academic insights to real-world applications, it can spark ideas for tailored implementations in a companyโs context. Moreover, exploring updated resources keeps professionals abreast of emerging technologies and trends, ensuring their strategies remain relevant and effective.
"For every action, there is a reaction," and in the world of data management, staying informed can mean the difference between making sound decisions and wasting resources.
Key Literature on Data Vault
- The Data Warehouse Toolkit by Ralph Kimball: This seminal work lays the groundwork for data warehousing concepts and methodologies, including the principles of Data Vault. It serves as a critical starting point for anyone looking to grasp data warehousing fundamentals.
- Building the Data Warehouse by William H. Inmon: Known as the father of the data warehouse, Inmonโs approaches and philosophies offer complementary insights to Data Vault strategies, especially regarding usability and design performance.
- Data Vault 2.0: Better, Simpler, Faster by Dan Linstedt: A must-read for practitioners, this modern take on the Data Vault method emphasizes its adaptability and advancements, specifically designed for todayโs data environments.
- Various online journals and white papers: Websites like Britannica host extensive articles and downloadable white papers that can provide additional insights into specific aspects of Data Vault implementations.
Resources for Mac Users
For professionals using Macs, the availability of specialized resources can significantly facilitate learning and implementation. Here are a few resources tailored to enhance the experience:
- Mac-specific forums on Reddit: Engaging with communities such as reddit.com can provide practical advice, insights from peer experiences, and support for troubleshooting specific problems related to Data Vault software on Mac systems.
- Data Vault community on LinkedIn: Following industry thought leaders and participating in discussions can keep users updated about new trends and software tailored for Mac users.
- Webinars and online courses: Platforms like Coursera or Udemy often host courses covering Data Vault techniques, many of which may include Mac-specific strategies for implementation.
- Vendor documentation: Always refer to the documentation provided by software vendors, as it often includes best practices, installation guides, and configuration tips relevant to macOS environments.
Staying informed through dynamic sources is key. Combining foundational texts with current conversations and resources specifically for Mac users enables an effective, agile approach to Data Vault implementation, serving the best interest of the organization.
Glossary of Data Vault Terminology
Understanding the specific terms associated with Data Vault is essential for anyone looking to deepen their knowledge and apply these methodologies effectively, especially for Mac systems. A glossary serves not just as a reference guide but as an essential tool that can clear up confusion, ensure consistency, and promote effective communication among teams. In a field as dynamic and nuanced as data warehousing, having a shared vocabulary can be the bedrock for collaboration and strategy execution.
A well-crafted glossary contributes to the following:
- Precision in Communication: When business decision-makers and technical teams engage, clarity in language can bridge the gap between different understandings of the same concept. This helps avoid misinterpretations that could derail projects.
- Efficiency in Training: For newcomers to the Data Vault methodology or even seasoned professionals switching over to Mac systems, a glossary provides a quick-reference guide that expedites the learning curve.
- Enhanced Documentation: Clear definitions and a comprehensive vocabulary help in creating better documentation, which is crucial for future reference and audits.
"In the digital age, language can sometimes be the only thread that connects various departments. A shared understanding of terms used in Data Vault can streamline workflows immensely."
Key Terms Defined
Here we define essential terminology that frequently appears in discussions around Data Vault, specifically tailored for Mac users:
- Data Vault: A specific approach to data warehouse modeling designed to offer a flexible and scalable architecture that accommodates changes without massive redesign.
- Hubs: Central entities that represent the core data from which everything else is derived. Hubs function as the access point to business keys.
- Links: These represent relationships between different hubs, capturing the context of how different pieces of data interrelate.
- Satellites: Used to store attribute sets related to the hubs. Satellites hold the descriptive data, allowing historical tracking and change management.
- Business Key: A unique identifier that denotes a specific entity in a business context. It's crucial in ensuring that the data is accurately retrieved and utilized.
- ETL (Extract, Transform, Load): A type of data processing that involves extracting data from various sources, transforming it into a usable format, and loading it into the data warehouse.
- Data Governance: A framework for managing data availability, usability, integrity, and security. Essential for maintaining compliance and quality.
These definitions can significantly impact how teams communicate and collaborate, driving more effective implementations of Data Vault on Mac systems and producing better business outcomes.