Analyzing Pricing Models in Data Science Services
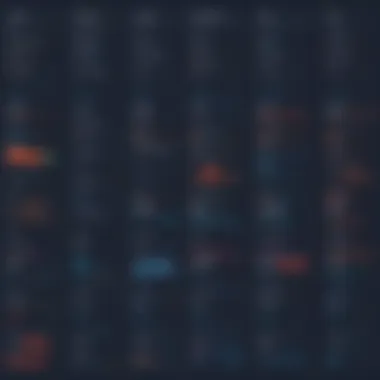
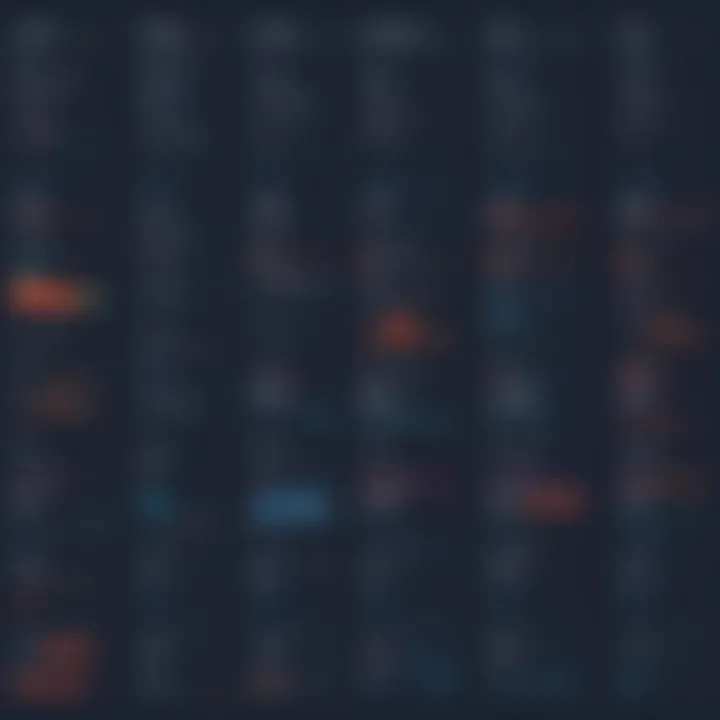
Intro
In today's data-driven era, gaining a competitive edge often hinges on effectively leveraging data science. However, understanding how much to invest in data-related services can be a daunting task. As businesses flounder through various pricing models, being able to discern the right choice becomes crucial. This article serves as a compass, guiding you through the intricate landscape of data science pricing structures. From dissecting the features inherent to these models to assessing the true value they can deliver, we’ll explore every nook and cranny, enabling you to make well-informed decisions.
Key Features
Overview of Features
Data science pricing models can vary significantly, catering to a range of business needs and project scopes. Below are some key features that commonly surface:
- Flexibility: Pricing models often allow adjustments based on project demands and resource availability.
- Scalability: Most solutions provide options that can grow alongside your business, ensuring that as data needs evolve, the pricing structure can accommodate expansion.
- Customizability: Tailored packages are frequently available to suit specific industry needs or particular business objectives.
"Understanding data science pricing can mean the difference between a good investment and a financial misstep."
Unique Selling Propositions
The distinctive advantages of specific data science pricing models often set them apart:
- Outcome-Based Pricing: Models that tie costs to project results can promote accountability and demonstrate value.
- Subscription Models: Ongoing support is typically provided, along with access to new features, helping businesses keep pace with technological advancements.
- Project-Based Pricing: Transparency in costs aids in budget planning, ensuring that financial expectations align with deliverables.
Pricing Models
Different Pricing Tiers
When diving further into data science pricing, businesses are likely to encounter several tiers depending on model selection:
- Freemium Models: Basic services are offered at no cost, enticing users to upgrade for more robust features.
- Hourly Billing: This traditional method calculates fees based on the number of hours spent on a project.
- Retainer Agreements: Clients pay a predetermined fee for a period in exchange for guaranteed access to data science expertise.
- Performance-Based Contracts: Costs are linked to specific deliverables or ROI, aligning provider incentives with client success.
ROI and Cost-Benefit Analysis
Before jumping into a pricing structure, it’s essential to assess potential returns. Engaging in a thorough ROI and cost-benefit analysis enables businesses to:
- Understand Value Delivery: Analyze what tangible benefits the data science services can yield.
- Make Concrete Comparisons: Evaluating multiple pricing models offers clarity on which provides the best bang for your buck.
- Long-Term Planning: Forecasting potential outcomes helps in budgeting effectively for future projects, capitalizing on data insights.
The Landscape of Data Science Pricing
Pricing in the data science field is not a one-size-fits-all situation. Each project brings with it unique challenges, expectations, and complexities that influence how services are priced. Building a firm grasp on this landscape is crucial for businesses that aim to expand their operations through data-driven insights. This understanding can significantly impact key business decisions, from budgeting to project planning and vendor selection. A well-informed approach allows stakeholders to negotiate effectively, align financial commitments with project goals, and ultimately ensure that they derive sufficient value from their investments in data science initiatives.
Preface to Data Science Pricing
Data science pricing is fundamentally intertwined with the intricate nature of the services provided. Unlike traditional market offerings, data science solutions often vary drastically based on project size, scope, and technical requirements. It’s not just about the hours worked or the resources expended—there exists a layered complexity stemming from the types of data used, the methodologies applied, and the desired outcomes.
In the data science arena, pricing models can be likened to tools in a craftsman's toolbox, each designed for specific tasks and outcomes. For instance, some pricing structures cater to shorter, well-defined projects, while others accommodate long-term engagements with evolving scopes. To navigate this terrain, it’s vital for decision-makers to grasp how these pricing models function in relation to their specific needs and objectives. By doing so, organizations can not only pinpoint the option that best aligns with their vision but also better allocate resources and manage expectations.
Importance of Understanding Pricing Models
Understanding the nuances of data science pricing models serves several essential purposes:
- Informed Investment Decisions: When businesses understand the different pricing structures, they can make more educated choices about where to allocate their resources. Awareness of potential costs can enable companies to set realistic budgets without overspending or compromising on quality.
- Risk Management: Different pricing models carry varying levels of risk. For instance, project-based pricing might lead to unpredictable costs if the scope expands unexpectedly, whereas retainer agreements can offer a more stable expense structure. Recognizing these factors ahead of time helps businesses better prepare for potential surprises.
- Value Proposition Clarity: Engaging with data science services can be a significant investment, but understanding pricing models helps validate that investment. Organizations can better gauge whether a pricing structure allows for performance measurement, ensuring that the value received is proportional to the costs paid.
"A well-defined pricing strategy arms businesses with the knowledge they need to maneuver the complex landscape of data science, transforming data into actionable insights."
The interplay of these models creates a busy marketplace—one filled with opportunity but also fraught with uncertainty. By carefully dissecting these pricing structures and their implications, businesses are better prepared to make decisions that will enhance their strategic goals and drive their success.
Common Pricing Models in Data Science
Understanding the landscape of data science pricing is essential for both businesses and professionals involved in this field. The pricing model selected can significantly influence project outcomes, budget allocations, and even the long-term relationships between clients and data scientists. In this section, we will explore four prevalent pricing models: hourly rates, project-based pricing, retainer agreements, and performance-based pricing. Each model comes with its own set of features, advantages, and downsides that companies should carefully consider when making decisions regarding their data science investments.
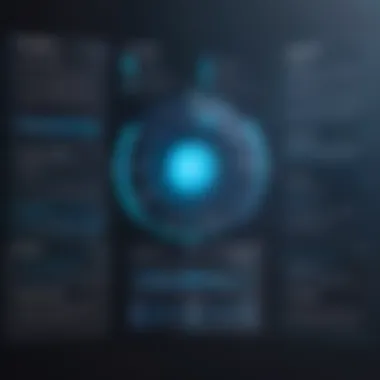
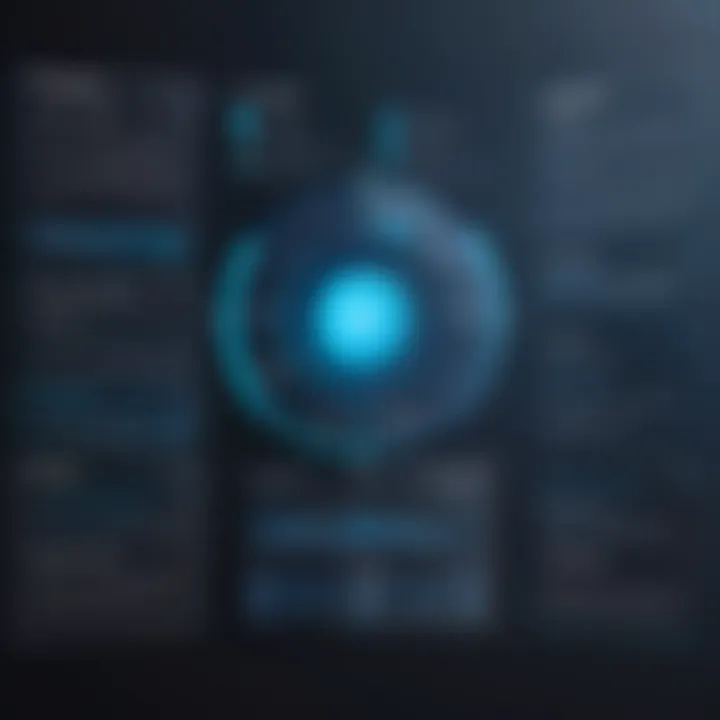
Hourly Rates
Hourly rates are among the most straightforward pricing models in data science. This method allows clients to pay for the actual time spent on a project, which can often appeal to those who are managing tight budgets or uncertain project scopes.
Advantages of Hourly Billing
The flexible nature of hourly billing is a significant advantage. When clients opt for this method, they pay only for the hours worked. This can be particularly useful in situations where project scopes are unclear or likely to change. Oftentimes, it allows both parties—the client and the data scientist—to re-calibrate expectations and budget as the project unfolds. Another positive aspect is that it often incentivizes productivity; the more efficient the data scientist is, the more they can maximize their revenue.
However, while hourly billing can be beneficial for both sides, it can introduce volatility in project costs, particularly if the client's needs evolve over time, leading to extended hours and potential frustration on both sides.
Disadvantages of Hourly Billing
On the flip side, hourly billing can create an environment where there's a mismatch in expectations. It's easy for clients to feel apprehensive, wondering whether they are being overcharged or if work is taking longer than it reasonably should. Additionally, fluctuating hours can complicate budget forecasting, which can be a headache for financial planning.
Project-based Pricing
With project-based pricing, a fixed fee is established for a defined scope of work. This can be especially appealing for projects where the goals are clear and deliverables are specific.
Defining Project Scope
Defining the project scope is crucial for successful project-based pricing. A clearly outlined scope helps eliminate ambiguity surrounding project deliverables, timelines, and costs. This creates a solid foundation for the working relationship and ensures that both parties are aligned on expectations. For many businesses, having a single price for an entire project assists in clear budget management and reduces the risk of unexpected costs.
However, developing a well-defined scope can be challenging. If the scope is not adequately detailed, clients risk entering agreements that lead to disputes or dissatisfaction if the final product does not meet expectations or if the project requires unforeseen adjustments.
Risks and Considerations
Project-based pricing, while desirable, carries certain risks. One pivotal risk is scope creep, wherein a client's needs evolve out of the initial project parameters, potentially affecting timelines and increasing costs if additional work isn't pre-negotiated. Therefore, it is crucial for both parties to maintain transparent communication and adjust agreements as necessary. This can be effective if both sides value flexibility and open dialogue.
Retainer Agreements
Retainer agreements provide a predictable and consistent billing process over an extended period. Instead of project-specific pricing, the client pays a regular fee for ongoing services or availability.
Benefits of Retainers
One of the most significant benefits of retainer agreements is the assurance of access to specialized skills without the commitment of a full-time hire. For businesses that require continual data science expertise, retainers can promote ongoing collaboration and deeper understanding of a client’s needs. This approach fosters a relationship that may lead to faster response times and a more tailored service experience.
But, there lies an issue: clients may feel locked into an arrangement if the services provided do not meet evolving business needs or if there is insufficient work to justify the retainer fee.
Limitations of Retainers
Despite their benefits, retainers have limitations. One of the primary downsides is that clients may underutilize the contracted hours, turning out to be a cost without benefit. Furthermore, if a retainer relationship becomes stagnant, it might be challenging to pivot to new projects or needs without renegotiating terms, which can waste both time and resources.
Performance-based Pricing
Performance-based pricing is a more results-oriented model. Under this approach, payments are linked to the success of outcomes based on predefined metrics.
How Performance Metrics are Established
Establishing performance metrics is a collaborative process that requires both parties to agree on criteria for success. These metrics can include aspects like project completion rates, key performance indicators (KPIs), or specific deliverables that must be met within a timeframe. This model incentivizes data scientists to deliver high-quality results that positively impact the client's bottom line, creating an environment ripe for shared success.
Nevertheless, clear communication is key. Performance metrics that are loosely defined can lead to misaligned goals and unmet expectations.
Challenges with Performance-based Models
While promising, performance-based pricing has its own complications. It may lead to stress for data scientists who feel the pressure to deliver exact results within tight deadlines. Additionally, external factors beyond the data scientist's control can influence project outcomes, which can create disputes if clients perceive that agreed-upon metrics have not been met.
Ultimately, the choice of pricing model involves balancing client needs, project requirements, and the specific skills of the data science team. Being flexible and transparent can mitigate many of the common pitfalls associated with each model, leading to more successful and satisfying outcomes for both sides.
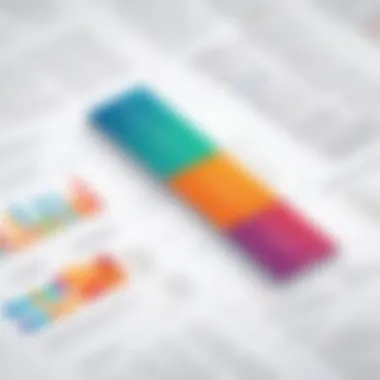
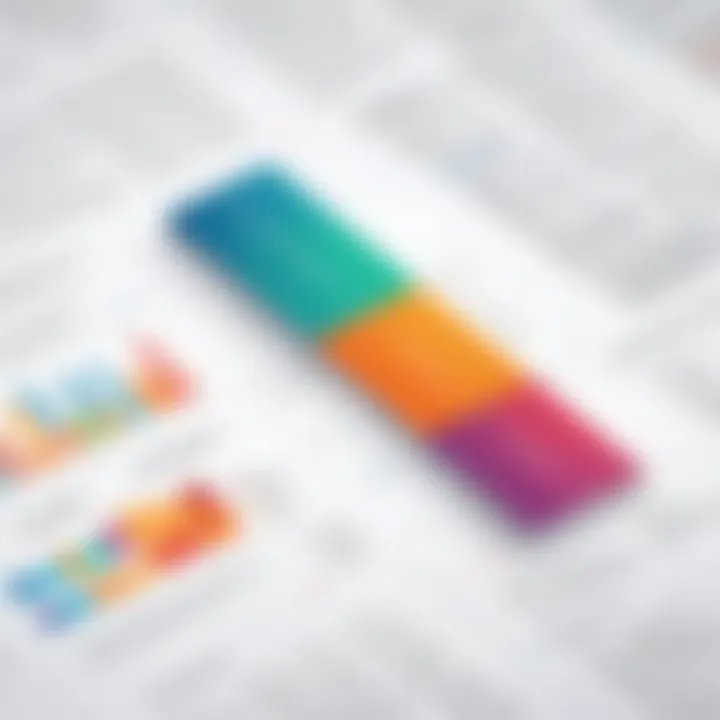
“The right pricing model can not only align interests between clients and data scientists but also foster stronger partnerships based on transparency and mutual understanding.”
Factors Influencing Data Science Pricing
Understanding the complexities of pricing in data science isn't just a matter of picking figures out of thin air. It’s a deep dive into various factors that shape what businesses will ultimately pay for services. Several key elements come into play here, each intricately connected with the nature of projects, expertise required, market dynamics, and data specifics. Analyzing these factors can aid companies not only in setting budgets but also in negotiating better deals that make financial sense in the long run.
Complexity of the Project
The project’s complexity plays a vital role in determining the cost. If the undertaking is straightforward, like basic data cleaning or simple visualizations, a lower price might be expected. However, when it starts involving intricate algorithms, machine learning models, or has layers of variables and dependencies, costs naturally escalate.
"Complex projects require not just time but also a level of skill and understanding that directly pushes the price upward."
Moreover, projects that need extensive cross-disciplinary integration—such as combining data engineering, data science, and even principles of user experience—are likely to reflect a higher price tag. Every added layer of expertise or hours spent doing work not initially accounted for adds to the complexity and, consequently, the pricing.
Expertise and Experience
How experienced and skilled a data scientist or team is can bump up the pricing as well. A well-versed data professional with a strong track record and proven results will charge more than a novice just starting out. For instance, say you’re searching for insights within healthcare analytics. An expert with experience in healthcare data regulations and biases would carry more weight than a generalist.
The experience translates into added value, as it can significantly speed up the time to complete a project by reducing back-and-forth communications and missteps. A solid reputation instills confidence, allowing for higher rates to be justified in clients' eyes.
Market Demand vs Supply
The interplay between supply and demand is a classic economic principle with direct implications for pricing in data science. When the demand for data science services soars—like during the waves of digital transformation—the prices tend to rise. On the other hand, when there’s an oversupply of data scientists due to a surge in graduates entering the field, prices may soften.
Businesses need to stay attuned to the market dynamics. For example, if a particular technology becomes the new trend, suddenly there's a rush to acquire data scientists proficient in that area. This surge can significantly influence the pricing model at play. Understanding local market trends is equally important as it can vary significantly from one geographic area to another.
Data Volume and Quality
The volume and quality of the data involved cannot be overlooked when discussing data science pricing. Working with large datasets can introduce challenges of processing and cleaning, impacting the overall project timeline and thus cost. Poor quality data that requires extensive wrangling often leads to increased hours dedicated to the project.
Additionally, higher quality data, which often provides clearer insights, tends to lead to more efficient analyses. Clients need to consider whether they are offering rich, clean datasets or if they are dumping raw, messy data on data scientists. The latter comes with a higher processing cost.
In summary, pricing in data science is not a one-size-fits-all affair. Each project brings its own set of conditions and costs are influenced by a matrix of variables. Recognizing these influencing factors empowers businesses to budget competently and engage in informed negotiations, unlocking the potential for long-term value from their investments.
Comparative Analysis of Pricing Models
In the labyrinth of data science pricing, a comparative analysis serves as a compass for businesses navigating their choices. This aspect is pivotal because it not only sheds light on the intricacies of different pricing structures but also helps stakeholders understand which model aligns best with their unique needs. By undertaking such an analysis, companies can develop a clearer picture of projected costs, the value received, and the implications of each approach.
There’s more than just a simple calculation of expenses; factors like project complexity, team expertise, and market fluctuations play a significant role in determining the overall financial impact. Therefore, a thoughtful comparison of models is indispensable for informed decision-making.
Cost-Effectiveness of Various Models
When evaluating the cost-effectiveness of data science pricing models, it’s essential to slice through the surface. Different models may appear appealing at first glance, but a deeper analysis often reveals the hidden costs that can surprise any unprepared business owner.
- Hourly Rates might boast flexibility, but they can spiral out of control if the project takes longer than expected, leading to unforeseen budget overruns.
- In contrast, Project-based Pricing typically offers a clearer financial roadmap. However, there’s a risk: if the initial scope isn’t well defined, these agreements can quickly become a double-edged sword.
- Retainer Agreements are often seen as a cost-effective option for ongoing support, but they necessitate a level of commitment that not every business is ready for.
- Lastly, Performance-based Pricing can align incentives, yet it relies heavily on the establishment of clear and measurable outcomes; if those aren’t set properly, it could end up being a costly gamble.
To encapsulate this, consider weighing these variables:
- Projected longevity of the project: Longer engagements might benefit from a flat rate.
- Scope clarity: Well-defined projects minimize the risk of excess costs.
- Quality of deliverables: Cheaper isn’t always better; sometimes, an investment upfront pays off later.
"Cost-effectiveness isn’t just about cheaper solutions; it’s about the right solutions that generate value over time."
Suitability for Different Business Needs
Every business has its own unique requirements, so selecting the right pricing model is akin to finding the right gear for a specific trek. It’s not one-size-fits-all. Considerations for suitability encompass several factors:
- Nature of the Project: If the scope is well understood and unlikely to change, a project-based model may suit perfectly. Conversely, if it's exploratory in nature, hourly rates could make more sense.
- Financial Stability: Startups might shy away from retainers, looking for hourly rates instead to manage cash flow; established firms often have the luxury of upfront investments.
- Urgency and Flexibility Needs: For businesses in a pinch, performance-based pricing can offer quick engagement but requires precise management.
- Expertise Requirements: High-expertise projects might justify the potential higher costs associated with certain models, while simpler projects benefit from more affordable structures.
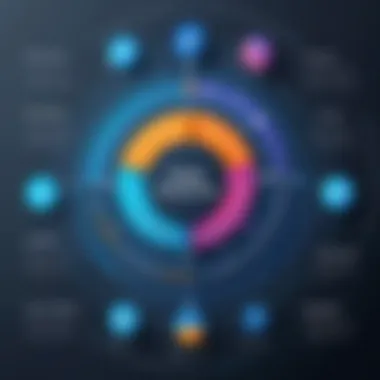
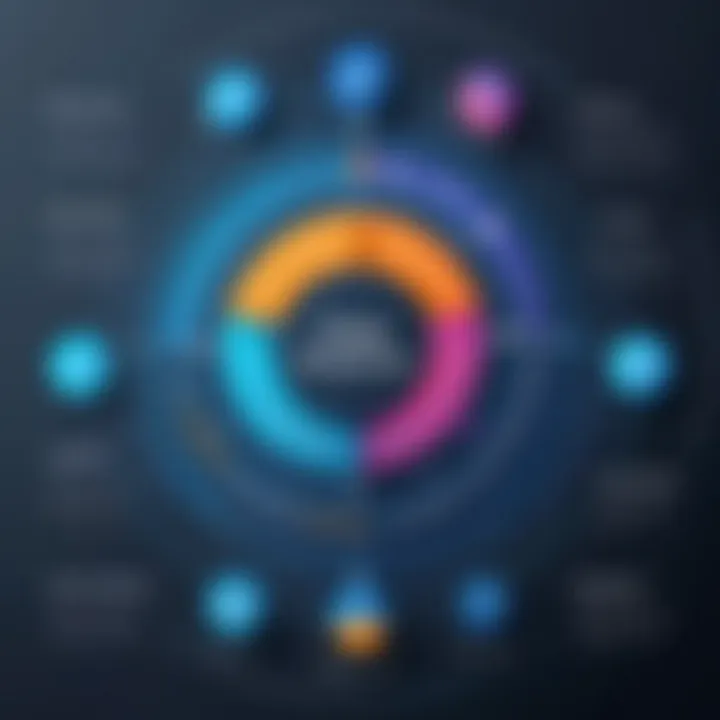
Budgeting for Data Science Projects
In the field of data science, budgeting extends beyond simple financial planning. It’s a blend of forecasting, strategic planning, and resource allocation. Understanding how to budget effectively for data science projects is essential for businesses, as it directly influences project success and return on investment. A well-planned budget can empower organizations to navigate the often complex and, at times, unpredictable landscape of data science.
Budgeting for data science projects requires a strong grasp of several specific elements:
- Detailed Cost Estimates: Data science projects often involve multiple components such as data acquisition, infrastructure, and personnel. Each of these elements needs to be accounted for in the budget. For example, if you're planning a predictive modeling project, knowing how much to allocate for acquiring datasets is crucial.
- Resource Management: It's not just about money; it's also about effectively managing human resources. Having skilled data scientists, engineers, and analysts can significantly impact your project’s trajectory. You have to evaluate their costs against the value they bring.
- Risk Management: As with any project, risks lurk around every corner. Establishing a buffer in your budget for unexpected costs can save you from potential headaches down the road. A well-thought-out risk management strategy can help keep your project under budget and on track.
Estimating Costs Accurately
Cost estimation is the backbone of effective budgeting. For data science projects, various factors come into play, and an accurate estimate can pave the way for success.
- Break Down the Components: Start by disassembling the project into components. If you are working on a machine learning model, consider costs tied to data collection, cleaning, model training, and deployment separately. This helps to reveal where your money goes.
- Use Historical Data: If your organization has undertaken similar projects in the past, use this historical data as a benchmark. Consult with colleagues who've managed comparable initiatives to acquire insights about cost variances.
- Consider External Factors: Market rates for talent, software tools, and cloud resources can fluctuate. Ensure to account for current market conditions and potential changes during the project's lifespan.
- Iterative Refinement: Cost estimates need not be static. They can be refined as you gain more knowledge about the project. An initial rough estimate can help guide your early budget, but be prepared to adjust as more data becomes available.
Managing Unexpected Expenses
Despite the best planning, unexpected expenses are a reality in data science projects. The ability to manage these costs efficiently is what separates successful projects from those that falter.
- Establish a Contingency Fund: It is prudent to allocate a portion of your budget—typically between 10 to 20 percent—specifically for unanticipated expenses. In data science, this could cover additional software licenses or unexpected infrastructure needs.
- Maintain Flexibility: Projects evolve. New insights might lead to changes in scope, requiring adaptability in your budgeting process. Your ability to pivot when faced with new challenges can mitigate the impact of unforeseen costs.
- Transparent Communication: Keeping stakeholders informed about potential financial pitfalls can help set realistic expectations. When everyone understands that unexpected expenses can arise, it creates a collaborative atmosphere to address them.
Budgeting isn’t just about limiting expenses; it's about empowering your team to navigate uncertainty and still achieve impactful outcomes.
Understanding the nuances of budgeting for data science projects is vital for any organization looking to leverage the power of data effectively. By focusing on accurate cost estimation and managing the unexpected, businesses can not only improve their decision-making but also increase the likelihood of project success.
Future Trends in Data Science Pricing
Understanding the future trends in data science pricing is crucial for businesses navigating a landscape that's constantly evolving. As technology advances and market demands shift, organizations need to stay informed about how these changes influence their budgeting and investment strategies in data science services. Companies are not just looking for immediate results; they also want to ensure long-term sustainability and growth. By grasping these emerging trends, decision-makers can make selections that lead to better performance and more strategic allocation of resources.
Emergence of Subscription Models
In recent years, there's been a noticeable shift toward subscription-based pricing in various sectors, and data science is no exception. This model offers businesses the flexibility to scale services according to their specific needs without the hefty upfront costs associated with traditional project-based pricing.
- Predictable Costs: Subscription models allow for easier budgeting as costs remain consistent over time. This predictability is beneficial for organizations looking to manage cash flow effectively.
- Access to Continuous Innovation: Subscriptions often include regular updates and new features. Users get the benefit of cutting-edge tools without needing to purchase new software or hire additional staff.
- Flexibility: Organizations can easily adjust their subscription plans to accommodate changing needs, enabling them to stay agile in a fast-paced market.
However, businesses must be aware of the nuances involved, such as committing to long-term contracts and ensuring that the service quality meets expectations over time. As more firms leverage this pricing model, understanding the competitive advantages and potential pitfalls becomes essential.
Impact of AI on Pricing Structures
AI is reshaping many aspects of business operations, and pricing structures in data science are no different. The integration of artificial intelligence into pricing strategies offers notable benefits, but also presents several considerations that businesses should keep in mind.
- Dynamic Pricing Strategies: AI tools can analyze vast amounts of market data to help refine pricing models. This capability allows businesses to adjust their prices in real-time based on demand fluctuations, competitor pricing, and even customer preferences.
- Personalization: AI can help create more tailored pricing models that align with individual client needs. This means that businesses could potentially offer more competitive pricing, which can enhance customer satisfaction and loyalty.
- Predictive Analytics: Leveraging AI improves forecasting accuracy, enabling companies to anticipate future pricing trends more effectively. Engaging with these insights can help organizations stay ahead in a competitive marketplace.
Although the potential for AI to enhance pricing strategies is substantial, businesses should remain vigilant. Relying too heavily on automated systems can lead to oversights, such as missing out on valuable qualitative insights that human decision-makers can provide. Therefore, a balanced approach, combining both AI-driven analytics and human expertise, is advisable for optimizing pricing strategies in data science.
The future of data science pricing will likely hinge on the successful integration of new business models, like subscriptions, alongside advanced AI technologies that refine how companies approach budgeting and investment.
Epilogue
Summary of Key Insights
To sum it up, the article has outlined several pivotal insights:
- Variety of Pricing Models: The landscape encompasses hourly rates, project-based pricing, retainers, and performance-based pricing, each serving different needs.
- Factors Influencing Costs: Project complexity, expert experience, market demand, and data quality act as essential determinants influencing pricing strategies.
- Future Innovations: With the rise of subscription models and the impact of AI, future pricing mechanisms will likely evolve, driving cost management and quality enhancement.
These facets not only highlight the importance of selecting the right pricing model but also stress the ongoing evolution within the field of data science. As businesses absorb these key insights, they can better align their budgets with strategic objectives.
Recommendations for Businesses
For businesses stepping into the data science realm, consider the following recommendations:
- Conduct Thorough Cost-Benefit Analyses: Understand the projected return on investment versus initial costs. Each pricing model carries distinct implications, which must resonate with company objectives.
- Choose Wisely Based on Needs: Look beyond the surface of cost. Align the pricing model with the complexity of your project and the desired outcomes. Consider retainers for ongoing, evolving needs, while project-based pricing might suit one-off analyses well.
- Stay Informed About Market Trends: Engage with resources like Wikipedia, Britannica, and communities on Reddit to keep abreast of changes in pricing and data science evolution. Awareness of emerging trends can provide a competitive edge.
- Build Strong Partnerships with Data Scientists: Trust and communication are vital. Collaborate closely with data scientists to ensure transparent discussions around pricing and expectations.
"The right pricing model can make the difference between a successful project and a financial pitfall. Pay close attention to the nuances and adapt accordingly."